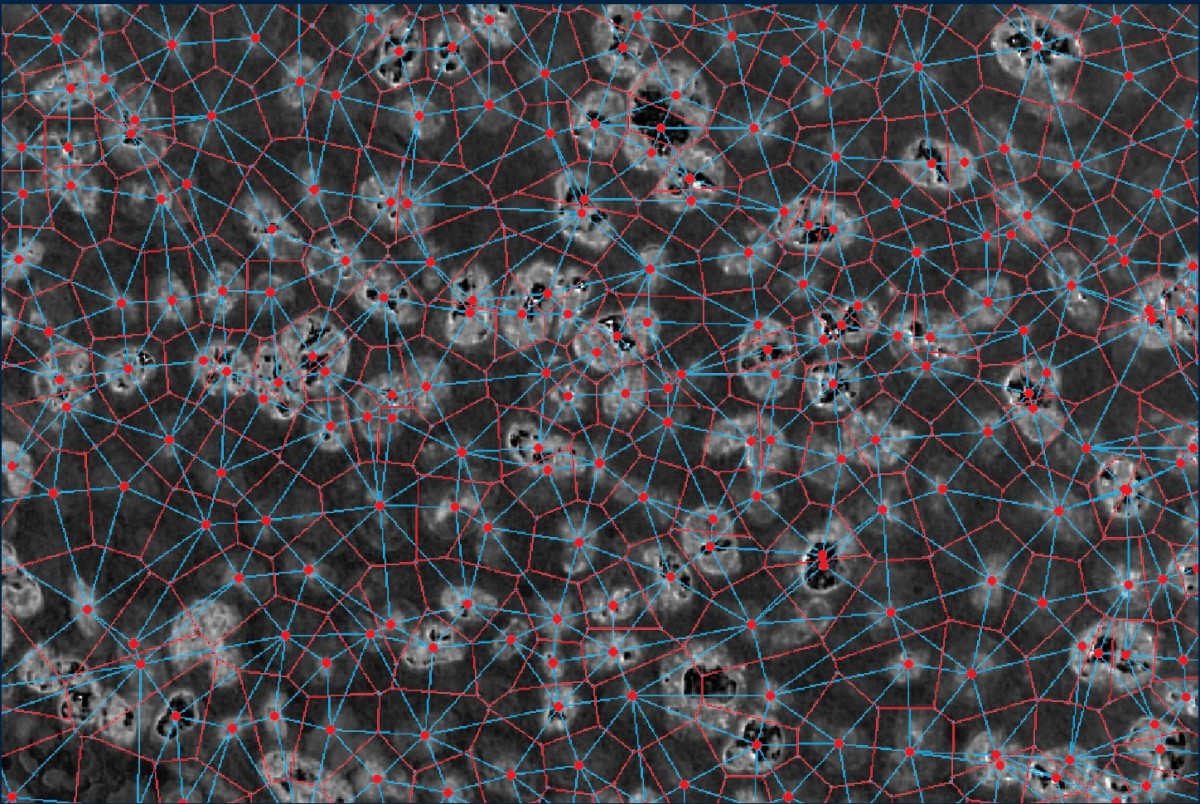
- Abstract
- Information in Pathology
- Biological Information
- Morphology and Function
- Genotype and Phenotype
- Information Analysis of Histological Slides
- Object oriented Image Information
- Application of Nyquist’s Theorem on Object oriented Information
- A Survey of Sampling Procedures
- Texture Oriented Image Information
- Artificial Intelligence in Diagnostic Pathology
- References
Theoretical Background of Digital Pathology
MedUP VeterInary Surgery Update
Klaus Kayser
Virtual microscopy or the diagnostic work with a completely digitized glass slide opens an effective tissue-based diagnosis. Based upon the experiences of telepathology application it has already reached a promising level of technology. Machines equipped with appropriate magazines can scan a glass slide in a few minutes. Software tools that are embedded or closely linked to hospital information systems work with open standards. They act as nodes in a network that meets the challenges of globalization in diagnostic pathology.
Additional fields of diagnostic medicine such as live imaging techniques as well as therapeutic strategies can be implemented. The final system will probably act as integrated medical Grid trying to offer an efficient and high performance service for the patient.
Information in Pathology
The term “information” has been derived from the Latin word informare which can be translated 'create by teaching’. It is a potential or existing pattern of material or energy, which can be recognized by a dynamic (living) system and influences its further behavior. Information includes two important features, namely (I) a source (pattern, texture, model, etc.) and (II) an “understanding receiver”. In practice, additional important parameters are the constancy of recognition and a new constellation for the living system. In other words, information forces the “receiver” to acting in a certain direction, and thus diminishes its uncertainty. The “measure” of information is usually performed by application of Claude Shannon’s mathematical theory of communication, which has been published in 1948 [430,431]. This theory derives an “information measure” from the statistical distribution of individual signals. Frequent (or common) signals possess less information than rare events. For an individual biological system, the most significant required information is: How to stay alive? Within this general structure, at least three different sub-types of information are prerequisite: a) to detect the necessary energy sources (food), b) to avoid individual catastrophes (detect enimies), and c) to provide the continuation of the species (reduplicate). How does this principle reflect to a complex living system?
Biological Information
As stated above, information requires a “repeatable” distribution of structures or events, which have to be distinguishable from the “background”. The repetition of events is obviously needed for “learning”: To learn from an event, which does no longer exist in the future is of no use and a waste of energy or resources. The complexity of living organisms requires a spatially and temporally synchronized exchange of information at different levels. We are used to analyze these levels from the “microcosmos” to the “macrocosmos”, i.e., from the arrangement of macromolecules to synchronized organoid and cellular structures including electronic signals, followed by boundaries, that separate inner from outer spaces, and finally steered movements and behavior of a whole individual. At all these levels, information is acquired from external sources, internally stored, and finally released from two storage systems, a long-term stored “data bank” and a flexible dynamic data flow often in combination with suppressor functions.. At the level of molecular biology or genetics, the fixed (internal) information storage system is the (desoxy) ribonucleid-acid (DNA), at the complex level of psychiatry it is called memory. The dynamic data flows include exchange of ions and changes of three-dimensional structures at the level of molecular biology, and those of acoustic and visual information at the behavior level. Information restricts the “degrees of freedom” of, and, therefore, opens a “goal” for the biological system. The different “levels of information” assure the flexibility of the system, which is often realized in “feed back mechanisms”. From this point of view, measurements of biological information sources include the definition of “limits to estimate healthy persons” and aberrations to define the nature and extent of a disease. Characteristic examples are the serum sugar concentrations (diabetes mellitus), electrocardiograms (ECG arrhythmias, cardiac infarction, etc.), or DNA (flow) cytometric measurements to distinguish between benign and malignant diseases. The repetition of information structures is realized in curves (time repetition) and in organoid structures (space repetition). Naturally, time repetitions reflect to space repetitions too; however, often only at specific organoid “levels” (fiber contractions, ion channels, etc.). The correspondent “space related” examinations include mainly molecular biological techniques such as comparative genomic hybridization (CGH), genomic arrays, or syntactic structure analysis at a light microscopic level. The measurements of biological information require both a characterization of the information structure and its contribution to the future behavior of the system (restriction of freedom degrees). Of major interest is the “outcome” of the whole system, i.e., its general health condition. The smaller the involved structure the more interactions have to be taken into account. The interpretation of basic molecular pathology data need detailed knowledge of the clinical and individual aspects of the involved patient. From the mathematical point of view strategies how to handle small event-large parameter sets (bioinformatics), and how to compare different images are the challenge. Interestingly, the majority of mathematical procedures to be applied has been developed at the beginning and middle of the last century; however, only nowadays high-speed and well-organized computers can perform the necessary calculations. The molecular-biological information is created by space- and time related structures, commonly called morphology and function. What is their relationship?
Morphology and Function
Our environment seems to be embedded in a four dimensional space which is considered to act as a framework for all phenomena in nature. Physical laws regulate the correlation between the different independent dimensions, which can be subdivided into three congruent, non-oriented dimensions (space) and an oriented independent one (time). Functions are relations between time and space, and the functions can be time independent, i.e., only space related, for example field forces such as gravity, electromagnetic fields, etc., or-in addition-time-dependent, for example laws of irreversible thermodynamics. In order to “detect” a function, a certain “physical equivalent” must exist in at least one of the space-associated dimensions, for example a point, line, ring, ball, stone, plant, animal, etc. In living or other time-dependent systems these “arrangements” usually have a specific time-characteristic [103,245,256]. After a period of a close time-relationship they seem to stay “stable” or nearly time-independent, followed by a period of strict time-relationship with a finally disarrangement or decay of the system. The outer and inner arrangement of these space-time equivalents, and their position and composition in biological or living systems at a certain time is called morphology. As it is considered to be an expression of genetic information (genotype) it is also called phenotype. It can be visualized by techniques that “fix” the spatial arrangement at a certain stage of development (fixation). Specific visualization techniques (staining procedures) allow the recognition of biological important structures such as membranes, nuclei, proteins, DNA fragments, etc, which reflect to the informative behavior of the whole system. These techniques opened a door into the world of cellular arrangement in organs with normal or healthy tissues, and its disturbance under conditions of abnormal functions. Until now, especially abnormal tissue growth could not be treated without knowledge of the cancer morphology. This “conventional light microscopic world” has been expanded to sub-cellular structures by use of electron microscopy and scanning electron microscopy, and to functional stages by visualization of the expression of macromolecules in certain stages of cellular development or abnormal growth, i.e., by immunohistochemical and ligandohistochemical staining techniques and related molecular-biological methods such as in situ hybridization or chromosome banding techniques. At a first glance, the knowledge of the presence or absence of a certain biological structure seems to be sufficient for disease classification and subsequent treatment of the patient; however, already a short reflection to the interactive information processes at the numerous information levels displays a functional complexity which is very unlikely to result in a simple binary (yes-no) reaction of the system.
What is the fundamental informative relationship between the expression of a certain biological structure and the associated function? What is the reason that abnormal cellular or organ function can be recognized by disarrangement of the associated geometrical “sources”? In other words, why have the cells given up their normal appearance once they have started to loose their normal function? It seems justified to assume that all biological processes or functions have to follow the general physical laws present in our environment. In addition, living system belong to so-called thermodynamically open systems. These systems are characterized by exchange of free energy and heat or entropy with their environment, and may stay on a low level of entropy for a long time by import of free energy and export of the produced entropy. The exchange of information is just a different word to characterize this energy import and heat export. A spatial repetitive import and export reflects to a (nearly) identical information exchange within the same informative level, for example an organ or its functional compartment. A normal function characteristic of an organ is that at least a group of neighboring cells is nearly identical in their energy and entropy balance. Thus, they posses the same level of entropy and current of entropy. Their exported products are identical structures (for example molecules, daughter cells, etc.) and are then arranged in a regular manner, which is basically defined by the energy forces and position of the “stem” cells. This is a prerequisite of a correct information transfer at a higher level. A disturbance of the cellular function has to be associated with a different energy balance that will lead to an “irregular distribution of derived structures”. Even when the “products” of the cells with different functions remain still identical, their final spatial arrangement will become altered, as the “steering” mechanism (feedback) of the higher order structures is diminished [258,278,280]. The disarrangement increases, when, in addition, the products also differ. As a result, the regularity or symmetry of a tissue reflects the homogeneity of cellular function, and the status of this homogeneity can be derived from the structure analysis. Analyzing spots of functional disturbances such as cancer, the level of this disturbance can be estimated by the calculation of the total of energy or entropy, which has to be added to a normal structure to create an abnormal texture. The following equations can be derived using micro-state distances (structures) and the expression of normal field forces:
ES (MST) = -k * S{pk *ln(pk)}; (1)
pk = 1/(N-1) * [Σ Σ ( Δmik/Δmik)2}]
k = Σ(mmk/mk)2 = standardization constant
Δmik = microstate differences between neighboring events
(distance, mass, staining intensities, etc.)
mmk, Δmk = mean of microstates in the macrostate k.
Measuring only the cellular proliferation will result in:
Σ Σ{ ( Δmik/mmik)2} = Σ[(Δm/m)2 + (Δd/d)2]
and CE = d(ES)/dt * x(1/s) (2)
______________________
ES = structural entropy
Dm = difference of DNA content between neighboring cells
m = mean DNA content of cells under consideration
Dd = difference of distance between neighboring cells and mean cellular distance
d = mean cellular distance
CE = current of entropy
s = surface of the system under consideration (tumor)
It could be shown that the structural entropy and current of entropy are good estimators for the survival of patients with bronchus carcinoma. The details are given in [234,245,256,272,282,285].
Additional texture-associated features such as mean distance between neighboring cells, distance between neighboring cells of different cell types, for example tumor cells and lymphocytes, have been reported to posses biological significance in various types of lung cancer. Basically, the terms Dm and m include features of vertices that originate an informative structure (graph) at a certain information level, and the calculated structural entropy is a measure of information disturbance within the system.
In practice, the visual analysis of the arrangement (attributed graphs) of biological “units” is a close derivative of the deviation of normal function and information transfer. The analysis of tissue structures is, therefore, an important task in the diagnosis and treatment of human diseases, especially in cancer patients. These measurements can be routinely performed on a broad variety of features, such as nuclei (DNA content), proteins (immunohistochemistry), DNA sequences (FISH), or vessels. How do the different biological bricks interact or fit together in terms of information storage and release?
Genotype and Phenotype
It is generally accepted, that the main level of biological information reflects to the individual cells of an organism, and that the basic information at this level is stored in the DNA located in cellular nucleus. Dependent upon the needs of the cell, this information can be retrieved and transferred into action by specific DNA-RNA pathways. The final outcome is a set of proteins that create new structures by destroying existing ones or interactions. The structural appearance of a biological system is, therefore, closely associated with the information stored at the DNA level. This storage is distinct, and can be subdivided into individual units, called genes. The genotype describes presence, absence and structural arrangement of genes within the DNA molecule, the phenotype the morphology at the level of cellular arrangements. Although the phenotype is a derivative from the genotype, the numerous interactions in releasing genetic information and creating new structures prohibit an individual genotype-phenotype expression. To give an example, cancer is nowadays considered to be a disease of “gene-abnormalities”, and, in fact, numerous cancer types have been reported to be associated with genetic disturbances. These include over-expression of oncogenes or alterations of so-called suppressor genes. An increased risk of breast carcinomas has been observed in patients with a BRCA-1, BRCA-2 or BRCA-3 gene. The percentage of breast cancer in women carrying this gene has first been calculated to about 90%, other reports give a considerably lower risk of 70%-80% [69,101,133,150,156,186,191,220,298,373,416,477,516]. Other genes such as retinoblastoma gene or polyposis coli gene contribute to similar percentages to the corresponding cancer types [66,79,134,166,225,227,406,413,459,472,515,524]. The existence of specific abnormalities at the genetic levels does not necessarily imply the outbreak of a disease that is obviously close associated with genetic information. The diagnosis of cancer still remains a domain of phenotype and of two dimensional image analyses at light microscopic or cellular level. Independent from the influence of external factors, of genotype or phenotype, there is no doubt that any disease is associated with a disturbance of information exchange at various biological levels. Thus, the question arises: What kind of information is presented in a histological image? How can it be recognized and implemented into a tissue-based diagnosis?
Information Analysis of Histological Slides
The information content of light microscopy images obtained from conventionally stained glass slides is composed of two main compartments, namely a) object-associated information, and b) non-object associated information. The different information components that contribute to tissue-based diagnosis are shown in (table 1).
Table 1: Survey of frequently viewed biological objects, adequate objective, and field-of-view
Search for | Objective | Field-of-view(mm2) |
Nucleus | x40 and higher | 0.03 and lower |
Cell types | x20 and higher | 0.1 and lower |
Small vessels, bronchioles | x10 and higher | 0.55 and lower |
Glands, Vessels, Nerves | x4-x10 | 3.5–0.55 |
The detection and classification of object-associated information requires a “division” of the image into an object-related space (compartments), and a non-object-related space (background) [263,307,388,276,193]. The objects searched for are usually “abnormal” events (cancer cell nuclei, inflammatory cells, external material, etc.), i.e. objects which display unusual features or which are not present in the analyzed tissue under normal (healthy) circumstances. For example, they comprise cells with alterations in size or internal structures (virus infection), cancer cells, inflammatory cells, or external organisms (bacteria, parasites). The majority of “classic” diagnoses is based upon the detection and correct identification of these “objects”: A correct cancer diagnosis requires the correct and error-free proof of cancer cells, that of active tuberculosis the visualization of tuberculosis bacilli. The basic scheme of object-related diagnosis procedures is given in (figure 1). The first step is to divide the original image into an object and a background image. The second step analyses the objects in relation to their features (cellular and nuclear size, staining intensity, form factor, etc). Of major significance is the procedure of the object-background separation (thresholding), which can be object dependent or not [38].
Having identified the objects, their spatial arrangement can possess diagnostic information too, for example in specific growth pattern (granulomas, adenoid growth pattern, epidermoid cellular arrangements, etc.). These features can be analyzed by various techniques, for example by syntactic structure analysis [34,35, 231,234,263,284,310,314]. A related graph is constructed which represents the gravity centers of the objects (nodes), a neighborhood relationship (edges), and node/edge related attributes (distances, sizes, integrated optical density, etc.). The procedure allows the definition of new (higher order) objects, if statistical associations (or repeated geometrical figures) can be obtained [230,245,284,480]. The spatial arrangement of identified objects is called structure. The location of objects can be randomly distributed or might follow certain rules. These rules are common in healthy tissues and include cellular agglutinations, strict neighborhood conditions between different cell types or formation of regular patterns.
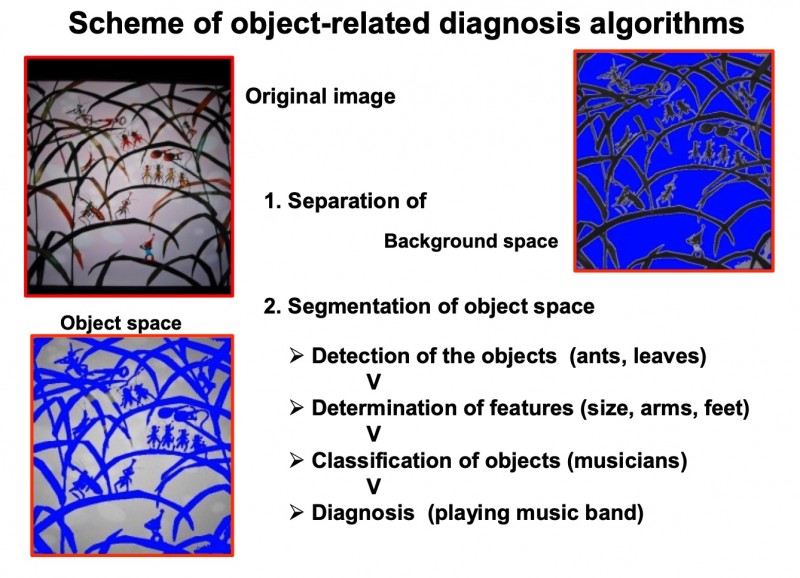
Fig. 1: Characteristics of object-related diagnosis algorithm in tissue-based diagnosis
In addition to the described procedures, non-object oriented information can be extracted from a histological image. The underlying representation of image information is usually called texture, and the procedure texture analysis [193,212,259, 314,457]. A texture is a gray value distribution, which might possess invariants in image transformation (symmetries). A texture can be analyzed by an autoregressive procedure that computes the gray values of pixels in relation to those of their neighbors. Similar, the same procedure can be applied to create images with artificial textures. A reproducible and invertible texture analysis results in a set of 5–6 parameters, and is, therefore, an appropriate tool to compute “similarities” between different images. It can also be used to transform an image into a two dimensional matrix and to compare images with known textures to those under diagnostic examination [193]. An example of the technique is given in (figure 2).
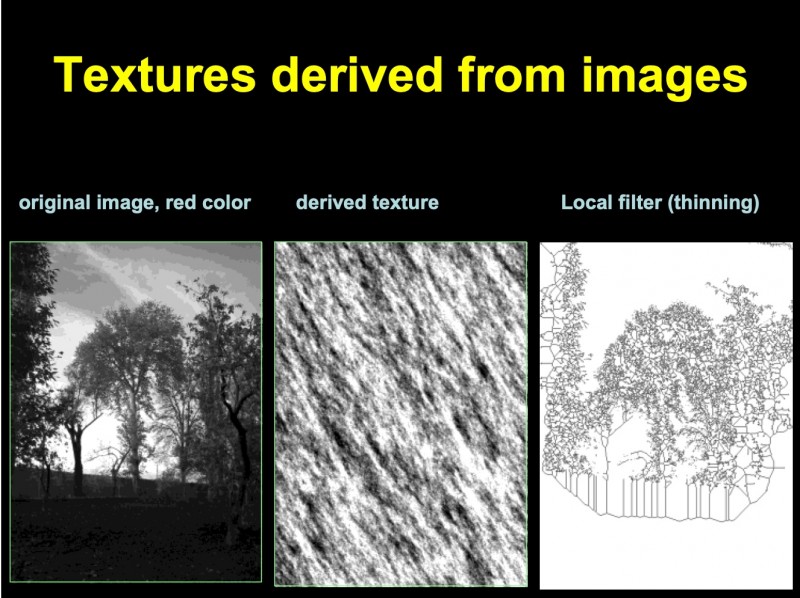
Fig. 2: Example of textures derived from autoregressive and local gray value (thinning) algorithms
The application of both object and texture associated diagnosis procedures results in a data set that represents the image as a whole. Thus, these algorithms seem to be useful to analyze virtual slides, which represent complete digitized glass slides. However, the digitalization of a complete glass slide creates images measuring several GB in size [263,284 107,122,123,534]. Therefore, the question arises whether the diagnosis information content of the complete image can be extracted from included image compartments, and, if yes, what is the obtained accuracy, i.e. sensitivity and specificity?
Object oriented Image Information
Obviously, image information is addressed to a viewer (or observer), who will specifically react if she or he receives (can see) the displayed information. In principle, image information is related to certain area (or space) associated features that “can be recognized”. The displayed features permit to separate these areas from the other compartments of the image. The total of both compartments add up to the complete image without overlapping. The distinct “information possessing areas” are called “objects”, and the remaining part “background”. The features of an object define its appearance, and can be computed in gray values of distinct areas (pixels) that lie within and outside of the object. Naturally, distinct areas that contain different quantities and quality of information might be present. The algorithm to detect the image objects is, therefore, a prerequisite to seeing specific image information, and to react appropriately, for example, to state a diagnosis. The object detection method is called segmentation. The accuracy of object segmentation depends upon
- the size of the objects in relation to the total image size (area or volume fraction)
- the gray value differences of pixels that are located at the object boundary from those that are located in the surrounding neighboring image areas (gradient).
- the possibility to add external information to the segmentation procedure, and
- the applied segmentation procedure.
Naturally, the size of an object has to fit into the whole image to be correctly identified. In tissue-based diagnosis, the location of objects within the image
frame is given by random, i.e. cannot be predefined. Thus, the size of the field-of-view has to measure a multiple of that of the object to be identified. On the other hand, if the object size is too small in comparison to that of the view field, the object cannot be identified accurately, for example, by its size or internal gray value distribution. The reasons are the biological variation in size and features, and the decrease of gray value differences at the object boundary. Using a conventional microscope, the common magnification to identify objects in classic diagnosis ranges from 200:1 to 800:1.
The gray value differences between pixels at the object boundary and those of the surrounding “outside” play the main role in detecting and separating any “information area” from the background. The greater and the more homogeneous the difference the easier is the segmentation. Variations that occur due to biological variance or image noise can be smoothed down or bridged using adequate filters; however, only to a certain degree. In conventional tissue-based diagnosis, the segmentation is either performed in a certain color space (nuclei usually stain blue) or in a singular gray value space that is artificially created from all three color spaces by specifically chosen color transformation. A commonly used method is the so-called principle component analysis and applying the Karhune-Loewe expansion [309, 377 92]. To use, in addition, external image information sources to correctly identify an object is still under development in tissue-based diagnosis, and has not been broadly come into practice to our knowledge. The technological approaches focus on the application at the stage of image acquisition, and the so-called smart cameras have to be mentioned here [73 486 464].
The implementation of smart cameras does not rely on a centralized server, and might use peer-to-peer computing to perform the various steps in the gestalt recognition system across the network: so-called distributed smart cameras [89] The underlying principle describes a set of object features that are no longer related to the object as a whole. In contrast, the object itself is considered to act as the “whole image”, and internal compartments are selected that are characteristic of the complete object to a high degree. A characteristic example is shown in (figure 3). A shark can be recognized by its fin already if it is seen above the sea surface although the entire body of the animal is hidden in the water. The shape and size of the fin are characteristic for this animal. Thus, the general shape of the shark and its location can be derived solely from its fin. In tissue-based diagnosis, the only similar algorithms have been applied to “characterize” the image background in relation to detected objects. This example analyzes the number of proliferating tumor cells in association to their distance from the nearest neighboring vessel 228. Additional applications, for example detection of tumor cells in small biopsies, or artificial display of most likely tumor growth in areas outside the field-of-view have not been reported to our knowledge until now.
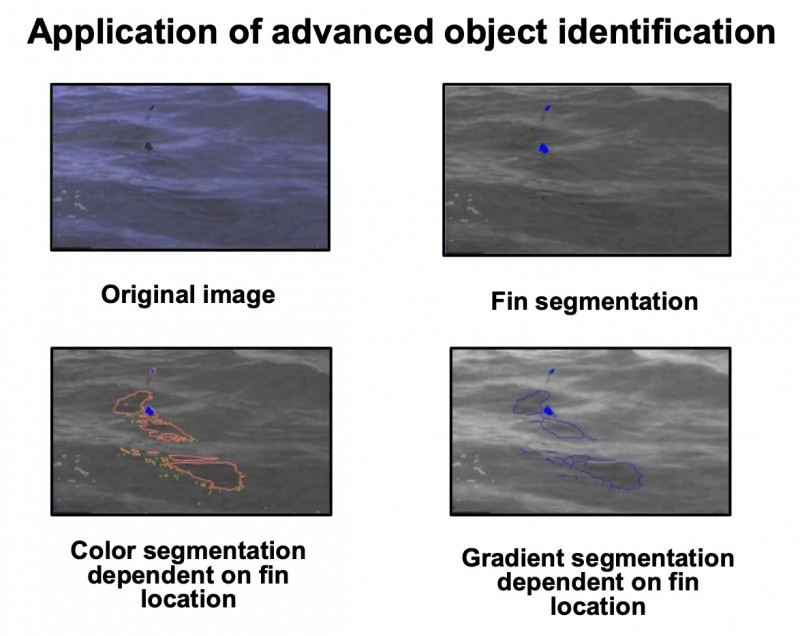
Fig. 3: Example of external information-related feature extraction. The shark can be identified by knowing the internal association between its different features (fin, body size, water)
Conversely, several scientists have investigated the most appropriate segmentation procedures [47,155,281,337,350,463]. Accurate object segmentation is the prerequisite for detection of any object-associated information. The segmentation procedures can be applied with or without prior image transformations (for example, eroding and dilating). They either use a fixed segmentation threshold or a dynamic one with a fixed gray value difference at the object boundary. The dynamic procedure can be applied to the whole range of gray values or only within predefined limits. Whether one of the described procedures is superior to the others, remains an open question. It probably depends on the image quality and the “nature” (appearance) of the objects to be identified. In tissue-based diagnosis, especially in identification of immunohistochemically stained objects the dynamic segmentation methods seem to be more appropriate since they take into consideration unavoidable variations in color and intensity [231,263,267].
The segmentation of objects is followed by the necessary next step of object identification, as not all non-background events belong to an information-related object class. The object-associated diagnosis includes all classes of diagnosis, which describe a momentary situation of the disease, for example adenocarcinoma, tuberculosis, angiitis, etc. They also include terms of molecular biology and molecular genetics, such as neuroendocrine positive tumors, or expression of the HER2-neu gene. Speed- and severity-associated features are usually not derived exclusively from object-based properties. They require additional analyses of spatial relationship between one or several sets of objects, and are, thus, texture-associated.
The efficient identification of diagnosis-relevant objects requires a “balance” between the necessity to identify an object without diagnostic errors, and the probability to “find” the corresponding objects within the field-of-view. What is a reliable solution, and how does it work?
Application of Nyquist’s Theorem on Object oriented Information
The image obtained from digitalization of a complete glass slide is called “virtual slide”. A virtual slide image file may be several GB in size [86,107,112,182,314,344,407]. It is generated by the acquisition of multiple image compartments that are “patched together” (patchwork procedure) [263,311,312,344,497,503]. The image acquisition time for a virtual slide ranges from minutes to hours, dependent, in part, on the desired image resolution. Once such a virtual slide image file has been acquired, it can be used for numerous purposes including image quantification, storage and retrieval in routine diagnostic work, steering source for automated tissue sampling in tissue micro arrays (TMA), continuous education, etc. The handling of such a very large data matrices, however, is not easy, and requires fast communicative connections and sophisticated programming. In addition to fast line connections and smart computer solutions, appropriate use of sampling procedures might be useful, might save time and avoid non-necessary efforts. One idea is based upon the principle of tissue-based diagnosis: Once the necessary information needed for diagnosis statement (and confirmation) has been detected, all additional investigations are stopped, i.e., the diagnostic procedure will be terminated immediately. For example, if tumor cells can be clearly identified in one or several image compartments, there may be no need to further analyze additional areas, (or the whole image), when further analysis will not affect the diagnosis.
The decomposition of an image into “diagnosis compartments” and their analysis might, therefore, improve the efficiency of a diagnostic procedure and further allow the calculation of the “risk” of missing an object with diagnostic significance. The risk calculation of object-associated diagnosis depends upon the object number and their size with relation to the sizes of the chosen compartments, as well as upon their sizes and number in respect to the size of the original image. If we consider the probability of an object diagnosis as “original diagnosis frequency” and the compartment division of the original image as “digitalization”, we can apply Nyquist’s theorem for an optimal adjustment of compartment size to the size of the complete image. According to Nyquist’s theorem the signal to be reconstructed must be sampled with a frequency at least two times greater than that to be reconstructed [263]. In other words, the number of pixels required to classify an object should amount to two (in a two dimensional space four) times more than the lower limit of recognition. Similar, the size of the “sampling space”, i.e. the diagnosis image compartment, must amount four times more than the pixel size of the objects divided by the relative frequency of objects present in the complete image. This assumption is useful for analysis of histological images, as these images usually contain connected tissue compartments.
A simple computation assuming that 10% of the original image (virtual slide) contain diagnostic significant objects, the size of an object measures 100 mm2, and an objective of *20 is required to identify the object results in a sample size 400 mm2, which should be repeated N = 1,.2. …10 times using randomly selected non-overlapping samples. If one of the samples contains an object, the procedure can be terminated. An overview of sample size useful for frequently diagnosed histological objects is given in (table 2).
Table 2: Survey of frequently viewed biological objects, adequate objective, and field-of-view
Search for | Objective | Field-of-view(mm2) |
Nucleus | x40 and higher | 0.03 and lower |
Cell types | x20 and higher | 0.1 and lower |
Small vessels, bronchioles | x10 and higher | 0.55 and lower |
Glands, Vessels, Nerves | x4-x10 | 3.5–0.55 |
A Survey of Sampling Procedures
The object-oriented information-extraction requires the identification of objects. The necessary algorithms can be applied to a histological image a) with or b) without additional spatial-associated predefined knowledge. This statement reflects to a random or non-random selection (sampling) of image compartments to be analyzed [231,263,267]. Basically, at least five different sampling procedures can be distinguished in the analysis of histological slides. They reflect to a) the aim of the image analysis, for example to evaluate the diagnosis information with the highest efficiency, b) to biological features or expected object properties, for example environment independent exhibition of receptors (visualization of macromolecules).
Random sampling does not require predefined information input, and is usually applied for measuring object properties, and the spatial distribution of objects within the tissue. Its counterpart is called stratified sampling, a procedure which either stops when identifying a wanted object (cancer cell), or preferable takes place in certain image areas (for example in close neighborhood to a vessel, at image compartments that display certain specific features, etc.). Both methods, i.e., random sampling and stratified sampling are object-oriented. They can be performed with a local independent (passive sampling) or local dependent (active sampling) object identification strategy. Active sampling procedures are often necessary in images that visualize macromolecule expression due to image features that are induced by laboratory conditions.
Finally, quite often “unknown” objects are identified that are difficult to be distinguished from artifacts. They are rare in frequency; might, however, belong to common objects, which express uncommon features (artifacts). The correct classification of these objects requires an event-and space-related identification of known surrounding objects, and is called functional sampling [231,267]. The application of stratified either active or passive sampling is most promising for automated extraction of diagnosis-oriented information from a histological image.
Texture Oriented Image Information
In contrast to object-related information, textures can be derived without the division of an image into a foreground (object space) and into a background. Unfortunately, an exact definition of a texture does not exist to our knowledge, neither in general nor in the context of image analysis. Most of the authors use the term “texture” for a general gray value function which can be derived from several repeating and “easy to see” basic image patterns. For example, according to Tamura et al. (1978) a texture can be defined by coarseness, contrast, directionality, line-likeness, regularity, and roughness [457]. Another, more practical and promising approach has been proposed by Voss and Süße [480]. The authors use an auto-regression function derived from the analysis of time sequences to describing and to creating textures. A six dimensional stochastic differential equation describes the correlation of random values (gray values) which are modified by associated coefficients. The basic algorithm is demonstrated in (figure 4). An example showing the original image, best fitting randomly computed objects and the calculated texture of a histological image (figure 5).
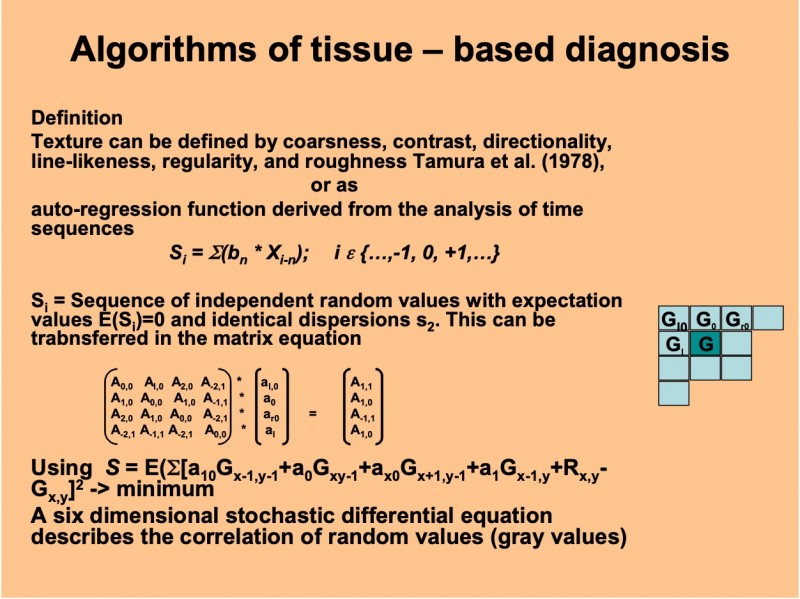
Fig. 4: Matrix presentation of the autoregressive texture analysis algorithm
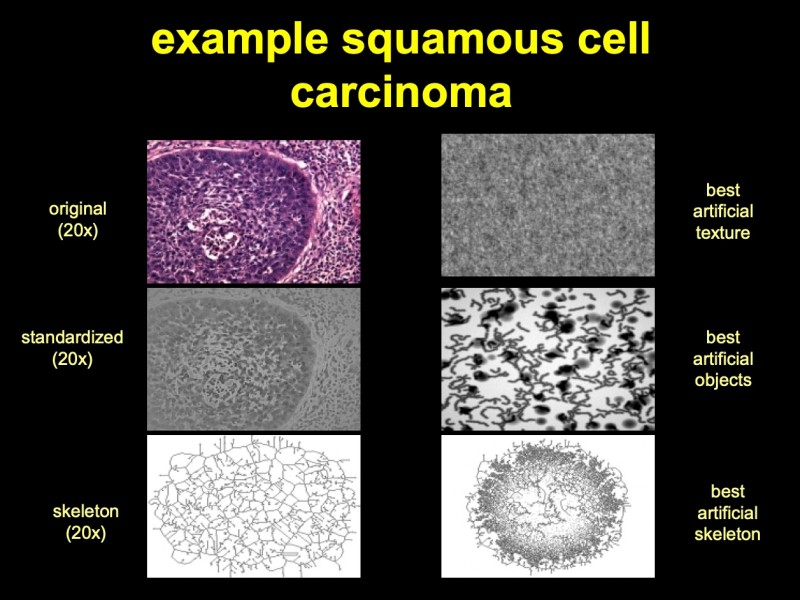
Fig. 5: Microphotograph of a squamous cell carcinoma of the lung (H & E, x20), its derived texture and best-fitting artificial texture and objects. The identification of objects and the analysis of textures are the two columns of information extraction from a histological image. Man is doing this by appropriate training and gathered experience. How can automatically a machine act in this manner? This machine obviously requires a certain amount of “intelligence”, commonly called artificial intelligence
The algorithm is basically dependent upon the image size; it becomes, however, quite independent for images of > 2,500 pixels in size (50 * 50 pixels) [263].
The texture synthesis using this auto-regression model and the corresponding derivation of textures from an image to be diagnosed permits a comparison of textures, and the computation of texture similarities. This idea seems be appropriate to determine useful diagnostic information based upon image textures.
Naturally, the idea of image analysis by auto regression algorithms is not limited to the original image, and can be applied to images that have undergone certain transformations of the original image too, such as linear and non-linear local filters (linear shift invariance filtering, Laplace, gradient filtering, etc.).
Reproducible texture analysis does not require an identification of objects, and is, therefore, not associated with object-related information. It is a second, independent approach to extract diagnosis relevant information from a histological image.
The identification of objects and the analysis of textures are the two columns of information extraction from a histological image. Man is doing this by appropriate training and gathered experience. How can automatically a machine act in this manner? This machine obviously requires a certain amount of “intelligence”, commonly called artificial intelligence.
Artificial Intelligence in Diagnostic Pathology
The term artificial intelligence has been defined by the American Association for Artificial Intelligence as follows: Artificial Intelligence (AI) is the understanding of the mechanisms underlying thought and intelligent behavior and their embodiment in machines. In principle, it is connected with image information acquisition, transfer and recognition. The term artificial intelligence was raised with the theoretical considerations about computer language and design, and dates back around 50 years to 1956. Since then, AI has made inroads into numerous sciences ranging from physic to philosophy, and from biology to logic. Its main practical application is seen in the realization of versatile industrial robotics; however, it is also applicable to solve questions such as: What is the rationale of a computer program? How can we transfer (visual, acoustic) information into a therapeutic act, i.e. automated diagnosis?
The simplest way to apply AI is the retrieval of previously codified information and the computation of potential associations. Therefore, in diagnostic pathology, AI was formerly involved the appropriate use suitable data bank and retrieval systems including automated language coding [157–159 161 247 378]. In parallel with the technical development of “computing machines” the contribution of AI to design and handling of these machines became more and more significant, and several hierarchically ordered information levels have been implemented. The reason of these strong interactions can be seen in the fact that AI is primarily a technique to understand and normalize any kind of decisions. On the other hand, decisions are based upon statistics. The larger the amount of available data the better is the statistical significance of a past or future decision. Any decision is closely associated with “information extracts of a data set” or-in terms of pathology-the diagnosis. A tissue-based diagnosis is only partly based upon image information. A general theory “how to reliably state a diagnosis based upon histological images” is unavailable to our knowledge; however, the following basic theorems can be given:
- Diagnosis finding is an algorithm that includes different levels of information. These levels reflect those related to the information transfer within a biological system (i.e., genotype, phenotype, symptoms, etc.).
- Their contribution to the final statement (diagnosis) depends upon the diagnosis itself. In other words, the performance of a diagnosis can be simulated by an autocorrelation function.
- The result is “feature-associated” information, and comprises, in addition, statements how to use this information (implicit instructions to the patient’s physician);
- A significant compartment of its parameters can be “extracted” from at least two different features of an image seen in a histological slide, namely from its texture (connective segmented areas), and its basic units (segmented disconnected areas).
- It includes a decision making process that is to a significant extent based upon image segmentation. In other words, the performance of “correct” image segmentation is the prerequisite for a “correct” diagnosis.
- All image “qualities” which are associated image segmentations will also influence the diagnosis.
- Finally, AI seems to provide adequate theoretical and technical methods to analyze the performance of image segmentation. Therefore, it should be able to be directly used for the assessment of tissue-based diagnosis.
Several publications describe and analyze the use of AI in decision making processes [34,36–38,33,155,203,205]. The applied techniques include Bayes decision formula, discriminate analysis, or neural networks. Usually, various information levels make contributions. The computation combines clinical data, such as sex, age, symptoms, with quantitative image data such as tumor cell grading, number of mitoses, or orientation of cell nuclei. The derived diagnosis commonly focuses on specific aims, for example, to analyze histopathological effects of androgen deprivation on prostate cancer [345,346]. The future development of AI in virtual slide technology will probably contribute in a different manner: It will be used to “analyze” variations of input data and their influence on the result. A characteristic aim is to search for image areas of interest for further diagnosis investigations [231,236,241,253]. The algorithm can be implemented in image acquisition systems, i.e. used to construct “smart image acquisition machines”, and to integrate image features (texture, objects) into final diagnosis decision systems [263]. Virtual slide technology can be used most effectively, if AI is implemented at various stages, starting from image acquisition, going via diagnosis assistance in search for specific areas, textures and elements, and finally approaching the definite diagnosis and its supervision by statistical estimations of probability, benefits and potential hazards of the stated diagnosis for the individual patient.
References
- Proceedings of the 1st European Symposium on Telepathology. Heidelberg, July 20-21, 1992. Zentralbl Pathol 1992, 138:381-434
- Telepathology. 2nd European Conference. Paris-Dijon, 9,11 June 1994. Proceedings. Arch Anat Cytol Pathol 1995, 43:189-304
- 4th European Congress on Telepathology. Proceedings of a symposium. Udine, Italy, June 19-20, 1998. Adv Clin Path 1998, 2:127-89
- The Age-Related Eye Disease Study system for classifying age-related macular degeneration from stereoscopic color fundus photographs: the Age-Related Eye Disease Study Report Number 6. Am J Ophthalmol 2001, 132:668-81
- Aas IH: Telemedicine and changes in the distribution of tasks between levels of care. J Telemed Telecare 2002, 8 Suppl 2:1-2
- Abadie JJ, Amardeilh MA, Delverdier ME: Immunohistochemical detection of proliferating cell nuclear antigen and Ki-67 in mast cell tumors from dogs. J Am Vet Med Assoc 1999, 215:1629-34
- Abbasi HR, Weigel R, Sommer C, et al.: Telepathology in neurosurgery. Stud Health Technol Inform 1999, 62:1-7
- Abeler VM, Danielsen HE: [Telepathology in Norway]. Tidsskr Nor Laegeforen 1999, 119:1335
- Ackerman AB: Are there too many dermatopathologists? Am J Dermatopathol 1985, 7:205-6
- Adachi H, Inoue J, Nozu T, et al.: Frozen-section services by telepathology: experience of 100 cases in the San-in District, Japan. Pathol Int 1996, 46:436-41
- Agarwal PK, Sharir M, Toledo S: Applications of parametric searching in geometric optimization. Proc 3rd ACM-SIAM Symp Disc Alg 1992:72-82
- Agha Z, Weinstein RS, Dunn BE: Cost minimization analysis of telepathology. Am J Clin Pathol 1999, 112:470-8
- Akervall J: Genomic screening of head and neck cancer and its implications for therapy planning. Eur Arch Otorhinolaryngol 2006
- Akiyama T, Teranishi Y, Nozaki K, et al.: Scientific Grid activities and PKI deployment in the Cybermedia Center, Osaka University. J Clin Monit Comput 2005, 19:279-94
- Allen EA, Ollayos CW, Tellado MV, et al.: Characteristics of a telecytology consultation service. Hum Pathol 2001, 32:1323-6
- Alli PM, Ollayos CW, Thompson LD, et al.: Telecytology: intraobserver and interobserver reproducibility in the diagnosis of cervical-vaginal smears. Hum Pathol 2001, 32:1318-22
- Almagro UA, Dunn BE, Choi H, et al.: Telepathology. Am J Surg Pathol 1998, 22:1161-3
- Andre S, Kojima S, Yamazaki N, et al.: Galectins-1 and -3 and their ligands in tumor biology. Non-uniform properties in cell-surface presentation and modulation of adhesion to matrix glycoproteins for various tumor cell lines, in biodistribution of free and liposome-bound galectins and in their expression by breast and colorectal carcinomas with/without metastatic propensity. J Cancer Res Clin Oncol 1999, 125:461-74
- Andre S, Unverzagt C, Kojima S, et al.: Neoglycoproteins with the synthetic complex biantennary nonasaccharide or its alpha 2,3/alpha 2,6-sialylated derivatives: their preparation, assessment of their ligand properties for purified lectins, for tumor cells in vitro, and in tissue sections, and their biodistribution in tumor-bearing mice. Bioconjug Chem 1997, 8:845-55
- Arber DA, Battifora H, Brynes RK, et al.: Certification and training in molecular pathology. Hum Pathol 1996, 27:430-1
- Asai T: Board certification of medical doctors and medical technologists in transfusion medicine in Japan. Transfus Apher Sci 2002, 26:115-9
- Auada A, Adam R, Leite NJ, et al.: Texture analysis of the epidermis based upon fast Fourier Transform in Sjögren - Larsson Syndrome. AQCH 2006, in press
- Aubele M, Burger G, Rodenacker K: Problems concerning the quality of DNA measurements on Feulgen-stained imprints. A study of five fixation techniques. Anal Quant Cytol Histol 1994, 16:226-32
- Bacon CJ: Debate on cot death. Standard system for postmortem examination and certification needs to be agreed. Bmj 2000, 320:310-1
- Bagarinao E, Matsuo K, Tanaka Y, et al.: Enabling on-demand real-time functional MRI analysis using grid technology. Methods Inf Med 2005, 44:665-73
- Balis UJ: Telemedicine and telepathology. Clin Lab Med 1997, 17:245-61
- Balogh AA, Preul MC, Laszlo K, et al.: Multilayer image grid reconstruction technology: four-dimensional interactive image reconstruction of microsurgical neuroanatomic dissections. Neurosurgery 2006, 58:ONS157-65; discussion ONS-65
- Baretton GB: [Certification of pathology institutions--a new direction? The view of a University Pathology Institute]. Z Arztl Fortbild Qualitatssich 2004, 98:727-32
- Barker G. Thousand Oaks, CA, Kennedy Western University, 2004, pp 1-205
- Barker G, McNeill KM, Krupinski EA, et al.: Clinical encounters costing for telemedicine services. Telemed J E Health 2004, 10:381-8
- Barker GP, Krupinski EA, McNeely RA, et al.: The Arizona Telemedicine Program business model. J Telemed Telecare 2005, 11:397-402
- Barker GP, Krupinski EA, Schellenberg B, et al.: Expense comparison of a telemedicine practice versus a traditional clinical practice. Telemed J E Health 2004, 10:376-80
- Barr M, McClellan S, Winokur T, et al.: An automated tissue preclassification approach for telepathology: implementation and performance analysis. IEEE Trans Inf Technol Biomed 2004, 8:97-102
- Bartels P, Weber J, Duckstein L: Machine learning in quantitative histopathology. Anal Quant Cytol Histol 1988, 10:299-306
- Bartels PH: Computer-generated diagnosis and image analysis. An overview. Cancer 1992, 69:1636-8
- Bartels PH: Automated primary screening devices. Expectations for the next generation. Acta Cytol 2000, 44:703-8
- Bartels PH, Bartels HG, Montironi R, et al.: Machine vision in the detection of prostate lesions in histologic sections. Anal Quant Cytol Histol 1998, 20:358-64
- Bartels PH, Montironi R, Duval da Silva V, et al.: Tissue architecture analysis in prostate cancer and its precursors: An innovative approach to computerized histometry. Eur Urol 1999, 35:484-91
- Bartels PH, Montironi R, Hamilton PW, et al.: Nuclear chromatin texture in prostatic lesions. I. PIN and adenocarcinoma. Anal Quant Cytol Histol 1998, 20:389-96
- Bartels PH, Montironi R, Hamilton PW, et al.: Nuclear chromatin texture in prostatic lesions. II. PIN and malignancy associated changes. Anal Quant Cytol Histol 1998, 20:397-406
- Bartels PH, Montironi R, Thompson D, et al.: Statistical histometry of the basal cell/secretory cell bilayer in prostatic intraepithelial neoplasia. Anal Quant Cytol Histol 1998, 20:381-8
- Bartsch G, Frick J, Rohr HP: Stereology, a new morphological method of study prostatic function and disease. Prog Clin Biol Res 1976, 6:123-41
- Bass SS, Cox CE, Reintgen DS: Learning curves and certification for breast cancer lymphatic mapping. Surg Oncol Clin N Am 1999, 8:497-509
- Batalis NI, Harley RA, Collins KA: Iatrogenic deaths following treatment for hypertrophic obstructive cardiomyopathy: case reports and an approach to the autopsy and death certification. Am J Forensic Med Pathol 2005, 26:343-8
- Bengtsson I: Geometrical Statistics - Classical and Quantum. Stockholm, 2005
- Bernroider G: Recognition and classification of structure by means of stereological methods in neurobiology. J Microsc 1976, 107:287-95
- Bibbo M, Kim DH, Pfeifer T, et al.: Histometric features for the grading of prostatic carcinoma. Anal Quant Cytol Histol 1991, 13:61-8
- Bjarkam CR, Pedersen M, Sorensen JC: New strategies for embedding, orientation and sectioning of small brain specimens enable direct correlation to MR-images, brain atlases, or use of unbiased stereology. J Neurosci Methods 2001, 108:153-9
- Black-Schaffer S, Flotte TJ: Teledermatopathology. Adv Dermatol 2001, 17:325-38
- Blobel GA, Moll R, Franke WW, et al.: The intermediate filament cytoskeleton of malignant mesotheliomas and its diagnostic significance. Am J Pathol 1985, 121:235-47
- Blosser SA, Zimmerman HE, Stauffer JL: Do autopsies of critically ill patients reveal important findings that were clinically undetected? Crit Care Med 1998, 26:1332-6
- Bock T, Svenstrup K, Pakkenberg B, et al.: Unbiased estimation of total beta-cell number and mean beta-cell volume in rodent pancreas. Apmis 1999, 107:791-9
- Bocking A, Giroud F, Reith A: Consensus report of the ESACP task force on standardization of diagnostic DNA image cytometry. European Society for Analytical Cellular Pathology. Anal Cell Pathol 1995, 8:67-74
- Bocking A, Giroud F, Reith A: Consensus report of the European Society for Analytical Cellular Pathology task force on standardization of diagnostic DNA image cytometry. Anal Quant Cytol Histol 1995, 17:1-7
- Boeryd BR: Experience with distant pathology demonstrations for clinicians in hospitals without local pathologists through the Swedish telepathology work station. Arch Anat Cytol Pathol 1995, 43:266-7
- Boesen AM: Stereologic analysis of the ultrastructure of isolated human T and non-T lymphoid cells. III. Studies in chronic lymphoid leukemias, hairy cell leukemia and some malignant lymphomas. Virchows Arch B Cell Pathol Incl Mol Pathol 1983, 43:165-78
- Bolender RP: Quantitative morphology for biologists and computer scientists: I. Computer-aided tutorial for biological stereology (version 1.0). Microsc Res Tech 1992, 21:338-46
- Borkowski W, Mielniczuk H: Telemedical management system of structured clinical documentation--application for quality assurance and multicenter clinical trials. Pol J Pathol 2003, 54:193-5
- Borkowski W, Mielniczuk H: [Use of medical record linkage in epidemiologic studies]. Przegl Epidemiol 2003, 57:553-9
- Bose R: Novel public key encryption technique based on multiple chaotic systems. Phys Rev Lett 2005, 95:098702
- Bosslet K, Luben G, Schwarz A, et al.: Immunohistochemical localization and molecular characteristics of three monoclonal antibody-defined epitopes detectable on carcinoembryonic antigen (CEA). Int J Cancer 1985, 36:75-84
- Brandan ME, Ruiz-Trejo C, Verdejo-Silva M, et al.: Evaluation of equipment performance, patient dose, imaging quality, and diagnostic coincidence in five Mexico City mammography services. Arch Med Res 2004, 35:24-30
- Brauchli K, Christen H, Haroske G, et al.: Telemicroscopy by the Internet revisited. J Pathol 2002, 196:238-43
- Brauchli K, Christen H, Meyer P, et al.: Telepathology: design of a modular system. Anal Cell Pathol 2000, 21:193-9
- Brauchli K, Helfrich M, Christen H, et al.: [The future of telepathology. An Internet "distributed system" with "open standards"]. Pathologe 2002, 23:198-206
- Brichard B, Heusterspreute M, De Potter P, et al.: Unilateral retinoblastoma, lack of familial history and older age does not exclude germline RB1 gene mutation. Eur J Cancer 2006, 42:65-72
- Britton M: The role of autopsies in medical audit: examples from a department of medicine. Qual Assur Health Care 1993, 5:287-90
- Brugghe J, Baak JP, Meijer GA, et al.: Rapid and reliable assessment of volume percentage of epithelium in borderline and invasive ovarian tumors. Anal Quant Cytol Histol 1998, 20:14-20
- Bryant H: Breast Cancer in Canadian Women. BMC Womens Health 2004, 4 Suppl 1:S12
- Bryant J: Telepathology as a substitute for traditional glass slides in a pathology consultation practice. Arch Dermatol 2002, 138:1380
- Bryant RJ, Banks PM, O'Malley D P: Ki67 staining pattern as a diagnostic tool in the evaluation of lymphoproliferative disorders. Histopathology 2006, 48:505-15
- Bunke H, Wang PS: Handbook of Character Recognition and Document Image Analysis. Singapore, World Scientific, 1997
- Burak O, Wayne W: A hierarchical human detection system in (un)compressed domains. IEEE Transactions on Multimedia 2002, 4:283-300
- Burthem J, Brereton M, Ardern J, et al.: The use of digital 'virtual slides' in the quality assessment of haematological morphology: results of a pilot exercise involving UK NEQAS(H) participants. Br J Haematol 2005, 130:293-6
- Burthem J, Brereton M, Ardern J, et al.: The use of digital 'virtual slides' in the quality assessment of hematological morphology: results of a pilot exercise involving UK NEQAS (H) participants. British Journal of Haematology 2005, 130:293 -6
- Burton JL, Underwood JC: Necropsy practice after the "organ retention scandal": requests, performance, and tissue retention. J Clin Pathol 2003, 56:537-41
- Buyya R: The Virtual Laboratory Project: Molecular modeling for drug design on Grid. IEEE Distributed Systems Online 2001, 2(5)
- Buyya R: The World-Wide Grid. http://wwwbuyyacom/ecogrid/wwg/ 2006
- Cabrera CM, Lopez-Nevot MA: APC and chromosome instability in colorectal cancer. Rev Esp Enferm Dig 2005, 97:738-43
- Cardillo MR, Di Silverio F, Gentile V: Quantitative immunohistochemical and in situ hybridization analysis of metalloproteinases in prostate cancer. Anticancer Res 2006, 26:973-82
- Carpenter AM: Stereology. Definition and historic background. J Histochem Cytochem 1979, 27:1535
- Carvalho PC, Gloria RV, de Miranda AB, et al.: Squid - a simple bioinformatics grid. BMC Bioinformatics 2005, 6:197
- Casanova H, Dongarra J: NetSolve: A network server for solving computational science problems. International Journal of Supercomputing Applications and High Performance Computing 1997, 11(3)
- Cass TA: Feature matching for object localization in the presence of uncertainty. Proc IEEE Conf Computer Vision and Pattern Recognition 1990:360-4
- Castleman K: Concepts in imaging and microscopy: Color image processing for microscopy. Biol Bull 1998, 194:100-7
- Catalyurek U, Beynon MD, Chang C, et al.: The virtual microscope. IEEE Trans Inf Technol Biomed 2003, 7:230-48
- Caulet S, Lesty C, Raphael M, et al.: Quantitative study of KI-67 antibody staining in non-Hodgkin's lymphomas using image analysis. Anal Quant Cytol Histol 1991, 13:279-87
- Cesarman E, Inghirami G, Chadburn A, et al.: High levels of p53 protein expression do not correlate with p53 gene mutations in anaplastic large cell lymphoma. Am J Pathol 1993, 143:845-56
- Chang H, Tiehan L, Wayne W, et al.: A peer-to-peer architecture for distributed real-time gesture recognition. Proceedings, International Conference on Multimedia and Exhibition, IEEE 2004
- Cheung AT, Donovan RM, Miller ME, et al.: Quantitative microscopy: I. A computer-assisted approach to the study of polymorphonuclear leukocyte (PMN) chemotaxis. J Leukoc Biol 1987, 41:481-91
- Chicken DW, Kocjan G, Falzon M, et al.: Intraoperative touch imprint cytology for the diagnosis of sentinel lymph node metastases in breast cancer. Br J Surg 2006, 93:572-6
- Chien J, Fu K: On the generalized Karhunen-Loewe expansion. IEEE Trans Information Theory 1967, IT13:518-23
- Clewley J: The Polymerase Chain Reaction for Human Viral Diagnosis. Boca Ratan, CRC, 1994
- Collan Y: Stereology in diagnostic pathology. Pathologica 1997, 89:462-6
- Coon JS, Deitch AD, de Vere White RW, et al.: Interinstitutional variability in DNA flow cytometric analysis of tumors. The National Cancer Institute's Flow Cytometry Network Experience. Cancer 1988, 61:126-30
- Cross SS, Burton JL, Dube AK, et al.: Offline telepathology diagnosis of colorectal polyps: a study of interobserver agreement and comparison with glass slide diagnoses. J Clin Pathol 2002, 55:305-8
- Crowley RS, Fujita K, Chavan G, et al.: Next-generation teaching systems using virtual slides. Virtual Microscopy and Virtual Slides in Teaching, Diagnosis, and Research. Edited by Gu J, Ogilvie RW. Boca Raton, Taylor & Francis, 2005
- Cruz-Orive LM, Gual-Arnau X: Precision of circular systematic sampling. J Microsc 2002, 207:225-42
- Cruz-Orive LM, Weibel ER: Sampling designs for stereology. J Microsc 1981, 122:235-57
- Cruz-Orive LM, Weibel ER: Recent stereological methods for cell biology: a brief survey. Am J Physiol 1990, 258:L148-56
- Dahiya R, Deng G: Molecular prognostic markers in breast cancer. Breast Cancer Res Treat 1998, 52:185-200
- Danilovic Z, Dzubur A, Seiwerth S: Concept of telepathology in Croatia. Arch Anat Cytol Pathol 1995, 43:282-4
- Danilovic Z, Seiwerth S, Kayser K, et al.: Experience based approach to interactive versus "store and forward" telepathology. Adv Clin Path 1998, 2:149-50
- David-Vaudey E, Burghardt A, Keshari K, et al.: Fourier Transform Infrared Imaging of focal lesions in human osteoarthritic cartilage. Eur Cell Mater 2005, 10:51-60; discussion
- de Macario EC, Macario AJ, Jovell RJ: Quantitative slide micro-immunoenzymatic assay (micro-SIA) for antibodies to particulate and non-particulate antigens. J Immunol Methods 1983, 59:39-47
- Dee FR, Heidger P: Virtual Slides for teaching Histology and Pathology. Virtual Microscopy and Virtual Slides in Teaching, Diagnosis, and Research. Edited by Gu J, Ogilvie RW. Boca Raton, Taylor & Francis, 2005, pp 111-40
- Dee FR, Lehman JM, Consoer D, et al.: Implementation of virtual microscope slides in the annual pathobiology of cancer workshop laboratory. Hum Pathol 2003, 34:430-6
- Della Mea V: Telepathology and other telemedicine fields: lessons to learn. Adv Clin Path 1999, 3:107-9
- Della Mea V: Telepathology and the Internet. Telemed Today 1999, 7:17-8, 44
- Della Mea V, Beltrami CA: Diagnostic telepathology through the Internet. Histopathology 1998, 33:485
- Della Mea V, Beltrami CA: Telepathology applications of the Internet multimedia electronic mail. Med Inform (Lond) 1998, 23:237-44
- Della Mea V, Beltrami CA: Current experiences with internet telepathology and possible evolution in the next generation of Internet services. Anal Cell Pathol 2000, 21:127-34
- Della Mea V, Cataldi P, Boi S, et al.: Image sampling in static telepathology for frozen section diagnosis. J Clin Pathol 1999, 52:761-5
- Della Mea V, Cataldi P, Pertoldi B, et al.: Combining dynamic and static robotic telepathology: a report on 184 consecutive cases of frozen sections, histology and cytology. Anal Cell Pathol 2000, 20:33-9
- Della Mea V, Cortolezzis D, Beltrami CA: The economics of telepathology--a case study. J Telemed Telecare 2000, 6 Suppl 1:S168-9
- Della Mea V, Forti S, Puglisi F, et al.: Telepathology using Internet multimedia electronic mail: remote consultation on gastrointestinal pathology. J Telemed Telecare 1996, 2:28-34
- Della Mea V, Puglisi F, Forti S, et al.: Telepathology through the Internet. J Telemed Telecare 1996, 2 Suppl 1:24-6
- Della Mea V, Puglisi F, Forti S, et al.: Expert pathology consultation through the Internet: melanoma versus benign melanocytic tumours. J Telemed Telecare 1997, 3 Suppl 1:17-9
- Della Mea V, Roberto V, Conti A, et al.: Internet agents for telemedicine services. Med Inform Internet Med 1999, 24:181-8
- Delta Mea V, Cataldi P, Pertoldi B, et al.: Dynamic robotic telepathology: a preliminary evaluation on frozen sections, histology and cytology. J Telemed Telecare 1999, 5 Suppl 1:S55-6
- Delvenne P, Delvenne C, Fridman V, et al.: [Prognostic evaluation of human solid tumors by immunohistology]. Rev Med Liege 1999, 54:677-82
- Demichelis F, Barbareschi M, Dalla Palma P, et al.: The virtual case: a new method to completely digitize cytological and histological slides. Virchows Arch 2002, 441:159-64
- Demichelis F, Della Mea V, Forti S, et al.: Digital storage of glass slides for quality assurance in histopathology and cytopathology. J Telemed Telecare 2002, 8:138-42
- Dervan PA, Wootton R: Diagnostic telepathology. Histopathology 1998, 32:195-8
- Descour MR, Kärkkäinen AHO, Rogers JD, et al.: Toward the development of miniaturized imaging systems for detection of pre-cancer. IEEE J Quantum Electronics 2002, 38:122-30
- Dierks C: Legal aspects of telepathology. Anal Cell Pathol 2000, 21:97-9
- Dietel M, Dierks C, Hufnagl P, et al.: [Automobile versus horse--immediate diagnostic section by telepathology]. Pathologe 2000, 21:391-5
- Dietel M, Hufnagl P: [Electronic communication in medicine]. Z Arztl Fortbild Qualitatssich 2001, 95:596-600
- Dietel M, Nguyen-Dobinsky TN, Hufnagl P: The UICC Telepathology Consultation Center. International Union Against Cancer. A global approach to improving consultation for pathologists in cancer diagnosis. Cancer 2000, 89:187-91
- Djemal KK: Document control and document management. Commun Dis Public Health 1999, 2:301-2
- Doolittle MH, Doolittle KW, Winkelman Z, et al.: Color images in telepathology: how many colors do we need? Hum Pathol 1997, 28:36-41
- Dorph-Petersen KA, Gundersen HJ, Jensen EB: Non-uniform systematic sampling in stereology. J Microsc 2000, 200 (Pt 2):148-57
- Dowsett M, Ellis MJ: Role of biologic markers in patient selection and application to disease prevention. Am J Clin Oncol 2003, 26:S34-9
- Doxey BW, Kuwada SK, Burt RW: Inherited polyposis syndromes: molecular mechanisms, clinicopathology, and genetic testing. Clin Gastroenterol Hepatol 2005, 3:633-41
- Drake LA, Goltz RW, Livingood CS, et al.: Recommendations for credentialing and privileging. Guidelines/Outcomes Committee. J Am Acad Dermatol 1998, 39:765-86
- Dunn BE, Almagro UA, Choi H, et al.: Use of telepathology for routine surgical pathology review in a test bed in the Department of Veterans Affairs. Telemed J 1997, 3:1-10
- Dunn BE, Almagro UA, Choi H, et al.: Dynamic-robotic telepathology: Department of Veterans Affairs feasibility study. Hum Pathol 1997, 28:8-12
- Dunn BE, Choi H, Almagro UA, et al.: Combined robotic and nonrobotic telepathology as an integral service component of a geographically dispersed laboratory network. Hum Pathol 2001, 32:1300-3
- Dunn BE, Choi H, Almagro UA, et al.: Telepathology networking in VISN-12 of the Veterans Health Administration. Telemed J E Health 2000, 6:349-54
- Dunn BE, Choi H, Almagro UA, et al.: Routine surgical telepathology in the Department of Veterans Affairs: experience-related improvements in pathologist performance in 2200 cases. Telemed J 1999, 5:323-37
- Dusserre P, Allaert FA, Dusserre L: Basic rules for the security of frozen section diagnosis through image transmission between anatomo-pathologists. Stud Health Technol Inform 1997, 43 Pt A:171-5
- Dzubur A, Danilovic Z, Caklovic N, et al.: A contribution to the quantitative analysis of transmitted images. Arch Anat Cytol Pathol 1995, 43:268-70
- Dzubur A, Seiwerth S, Danilovic Z: Benefits of image databank supporting the telepathology system. Adv Clin Path 1998, 2:158-9
- Eberlein-Gonska M: [Quality management and outcome--pathology sets an example]. Z Arztl Fortbild Qualitatssich 2004, 98:745-9
- Elias H: Stereology of parallel, straight, circular cylinders. J Microsc 1976, 107:199-202
- Elias H, Bokelmann D, Vogtle R: [Principles of stereology and morphometry presented with large intestinal carcinogenesis as example]. Verh Anat Ges 1976:911-6
- Elias H, Hyde DM: An elementary introduction to stereology (quantitative microscopy). Am J Anat 1980, 159:412-46
- Epstein JI, Amin M, Boccon-Gibod L, et al.: Prognostic factors and reporting of prostate carcinoma in radical prostatectomy and pelvic lymphadenectomy specimens. Scand J Urol Nephrol Suppl 2005:34-63
- Erlanson M, Casiano CA, Tan EM, et al.: Immunohistochemical analysis of the proliferation associated nuclear antigen CENP-F in non-Hodgkin's lymphoma. Mod Pathol 1999, 12:69-74
- Euhus D: Risk modeling in breast cancer. Breast J 2004, 10 Suppl 1:S10-2
- Falk S: [Certification of pathology institutions--a new direction? The view of a pathologist in private practice]. Z Arztl Fortbild Qualitatssich 2004, 98:733-7
- Farshid G, Pieterse S: Core imprint cytology of screen-detected breast lesions is predictive of the histologic results. Cancer 2006
- Farstad IN, Bofin A, Goplen AK, et al.: [Pathology--a changing discipline]. Tidsskr Nor Laegeforen 2000, 120:1796-801
- Fenner JW, Mehrem RA, Ganesan V, et al.: Radiosurgery planning supported by the GEMSS grid. Stud Health Technol Inform 2005, 112:190-7
- Ferrer Roca OF, Ramos A, Diaz Cardama A: Immunohistochemical correlation of steroid receptors and disease-free interval in 206 consecutive cases of breast cancer: validation of telequantification based on global scene segmentation. Anal Cell Pathol 1995, 9:151-63
- Figge MA, Blankenship L: Missense mutations in the BRCT domain of BRCA-1 from high-risk women frequently perturb strongly hydrophobic amino acids conserved among mammals. Cancer Epidemiol Biomarkers Prev 2004, 13:1037-41
- Flandrin G: [Strategy for remote diagnosis and the image bank. A specific application for telepathology for hematology]. Ann Pathol 1996, 16:155-8
- Flandrin G: Image bank, diagnostic codification and telediagnosis in hematology. Leuk Lymphoma 1997, 25:97-104
- Flandrin G, Valensi F, Macintyre E: An open thesaurus for diagnostic codification in practical hematology. Leuk Lymphoma 1997, 25:104-9
- Florentine BD, Wu NC, Waliany S, et al.: Fine needle aspiration (FNA) biopsy of palpable breast masses: comparison of conventional smears with the Cyto-Tek MonoPrep system. Cancer 1999, 87:278-85
- Foran DJ, Meer PP, Papathomas T, et al.: Compression guidelines for diagnostic telepathology. IEEE Trans Inf Technol Biomed 1997, 1:55-60
- Foster I, Kesselman C: Globus: A metacomputing infrastructure toolkit. International Journal of Supercomputer Applications 1997, 11:115-28
- Fu KS: Syntactic Pattern Recognition, Applications. Heidelberg, New York, Springer, 1977
- Gadaleta SJ, Paschalis EP, Betts F, et al.: Fourier transform infrared spectroscopy of the solution-mediated conversion of amorphous calcium phosphate to hydroxyapatite: new correlations between X-ray diffraction and infrared data. Calcif Tissue Int 1996, 58:9-16
- Gagnon M, Inhorn S, Hancock J, et al.: Comparison of cytology proficiency testing: glass slides vs. virtual slides. Acta Cytol 2004, 48:788-94
- Galiatsatos P, Foulkes WD: Familial adenomatous polyposis. Am J Gastroenterol 2006, 101:385-98
- Galizia G, Lieto E, Ferraraccio F, et al.: Prognostic Significance of Epidermal Growth Factor Receptor Expression in Colon Cancer Patients Undergoing Curative Surgery. Ann Surg Oncol 2006
- Gallo D, Zannoni GF, Martinelli E, et al.: Estradiol and phytoestrogens differently influence the rodent postmenopausal mammary gland. Menopause 2006, 13:72-9
- Galvez J, Howell L, Costa MJ, et al.: Diagnostic concordance of telecytology and conventional cytology for evaluating breast aspirates. Acta Cytol 1998, 42:663-7
- Gerdes J: Ki-67 and other proliferation markers useful for immunohistological diagnostic and prognostic evaluations in human malignancies. Semin Cancer Biol 1990, 1:199-206
- Germain C, Breton V, Clarysse P, et al.: Grid-enabling medical image analysis. J Clin Monit Comput 2005, 19:339-49
- Geusebroek JM, Smeulders AW, Cornelissen F, et al.: Segmentation of tissue architecture by distance graph matching. Cytometry 1999, 35:11-22
- Ghisleni G, Roccabianca P, Ceruti R, et al.: Correlation between fine-needle aspiration cytology and histopathology in the evaluation of cutaneous and subcutaneous masses from dogs and cats. Vet Clin Pathol 2006, 35:24-30
- Gibblin PJ: Graphs, Surfaces, and Homology. London, Chapman & Hall, 1977
- Girela E, Lachica E, Pounder D: Death certification of problem drinkers. Med Sci Law 1992, 32:233-6
- Giroud F, Delage B, Monet JD, et al.: Methodological considerations on Feulgen-staining applied to cells in primoculture: the model of osteoarthritic synovial cells. Anal Cell Pathol 1996, 11:1-12
- Giroud F, Haroske G, Reith A, et al.: 1997 ESACP consensus report on diagnostic DNA image cytometry. Part II: Specific recommendations for quality assurance. European Society for Analytical Cellular Pathology. Anal Cell Pathol 1998, 17:201-8
- Godwin TA: End of life: natural or unnatural death investigation and certification. Dis Mon 2005, 51:218-77
- Goh A: Cryptographic framework for document-objects resulting from multiparty collaborative transactions. Stud Health Technol Inform 2000, 77:1069-73
- Gombas P: Informational aspects of telepathology in routine surgical pathology. Anal Cell Pathol 2000, 21:141-7
- Gombas P, Skepper JN, Hegyi L: The image pyramid system--an unbiased, inexpensive and broadly accessible method of telepathology. Pathol Oncol Res 2002, 8:68-73
- Gombas P, Skepper JN, Krenacs T, et al.: [Past, present and future of digital pathology]. Orv Hetil 2004, 145:433-43
- Goncalves L, Cunha C: Telemedicine project in the Azores Islands. Arch Anat Cytol Pathol 1995, 43:285-7
- Goncalves L, Cunha C: Telepathology experiences in Portugal. Elec J Pathol 1995, 4:954-09
- Goodman JE, O'Rourke J (editors): CRC Handbook of Discrete and Computational Geometry. Boca Raton, CRC Press, 2004
- Goss PE: Breast cancer prevention--clinical trials strategies involving aromatase inhibitors. J Steroid Biochem Mol Biol 2003, 86:487-93
- Gribunov Iu P, Perov Iu L, Frank GA, et al.: [A method of determination of the accuracy of morphological diagnosis of surgical and biopsy material]. Arkh Patol 2004, 66:51-4
- Grimshaw A, Wulf W: The Legion vision of a worldwide virtual computer. Communications of the ACM 1997, 40
- Gu J: Recent advances in telepathology. Cell Vis 1998, 5:62-6
- Gu J, Ogilvie R, edts: Virtual Microscopy and Virtual Slides in Teaching, Diagnosis, and Research. Boca Raton, Taylor & Francis, 2005
- Gui GP, Hogben RK, Walsh G, et al.: The incidence of breast cancer from screening women according to predicted family history risk: Does annual clinical examination add to mammography? Eur J Cancer 2001, 37:1668-73
- Gundersen HJ: Stereology--or how figures for spatial shape and content are obtained by observation of structures in sections. Microsc Acta 1980, 83:409-26
- Gundersen HJ: Stereology of arbitrary particles. A review of unbiased number and size estimators and the presentation of some new ones, in memory of William R. Thompson. J Microsc 1986, 143 (Pt 1):3-45
- Gundersen HJ: Stereology: the fast lane between neuroanatomy and brain function--or still only a tightrope? Acta Neurol Scand Suppl 1992, 137:8-13
- Gundersen HJ, Bendtsen TF, Korbo L, et al.: Some new, simple and efficient stereological methods and their use in pathological research and diagnosis. Apmis 1988, 96:379-94
- Gundersen HJ, Jensen EB: The efficiency of systematic sampling in stereology and its prediction. J Microsc 1987, 147 (Pt 3):229-63
- Gundersen HJ, Jensen EB, Kieu K, et al.: The efficiency of systematic sampling in stereology--reconsidered. J Microsc 1999, 193:199-211
- Gundersen HJ, Osterby R: Optimizing sampling efficiency of stereological studies in biology: or 'do more less well!' J Microsc 1981, 121:65-73
- Guyton JR: Locational stereology performed within sampling fields in rat aortic wall, a polarized tissue. J Microsc 1984, 135 (Pt 1):103-14
- Halliday BE, Bhattacharyya AK, Graham AR, et al.: Diagnostic accuracy of an international static-imaging telepathology consultation service. Hum Pathol 1997, 28:17-21
- Hannon WH: Performance evaluation for screening laboratories of the Asia-Pacific region. Southeast Asian J Trop Med Public Health 2003, 34 Suppl 3:39-42
- Haroske G, Baak JP, Danielsen H, et al.: Fourth updated ESACP consensus report on diagnostic DNA image cytometry. Anal Cell Pathol 2001, 23:89-95
- Haroske G, Giroud F, Kunze KD, et al.: A telepathology based Virtual Reference and Certification Centre for DNA image cytometry. Anal Cell Pathol 2000, 21:149-59
- Haroske G, Giroud F, Reith A, et al.: 1997 ESACP consensus report on diagnostic DNA image cytometry. Part I: basic considerations and recommendations for preparation, measurement and interpretation. European Society for Analytical Cellular Pathology. Anal Cell Pathol 1998, 17:189-200
- Haroske G, Meyer W, Kunze D, et al.: Quality control measures for dna image cytometry in a telepathology network. Adv Clin Path 1998, 2:143-5
- Haroske G, Meyer W, Oberholzer M, et al.: Competence on demand in DNA image cytometry. Pathol Res Pract 2000, 196:285-91
- Harris G: Telepathology in Japan. Telemed Today 1999, 7:40-1
- Harris M, Hartley AL: Value of peer review of pathology in soft tissue sarcomas. Cancer Treat Res 1997, 91:1-8
- He L, Thomson JM, Hemann MT, et al.: A microRNA polycistron as a potential human oncogene. Nature 2005, 435:828-33
- Hedley DW, Clark GM, Cornelisse CJ, et al.: Consensus review of the clinical utility of DNA cytometry in carcinoma of the breast.Report of the DNA Cytometry Consensus Conference. Cytometry 1993, 14:482-5
- Helin HO, Lundin ME, Laakso M, et al.: Virtual Microscopy in Prostate Histopathology: Simultaneous Viewing of Biopsies Stained Sequentially With Hematoxylin and Eosin, and alpha-Methylacyl-Coenzyme A Racemase/p63 Immunohistochemistry. J Urol 2006, 175:495-9
- Höffgen H, Kayser K, Schlege lW, et al.: Low resolution structure analysis of corpus endometrium. Path Res Pract 1983, 178:131-4
- Hoschek W, Jaen-Martinez J, Samar A, et al.: Data management in an international data Grid project. Proceedings of the 1st IEEE/ACM International Workshop on Grid Computing (Grid'2000), Bangalore, India, 17-20 December 2000 2000, Springer: Berlin
- Howard V, Reid S, Baddeley A, et al.: Unbiased estimation of particle density in the tandem scanning reflected light microscope. J Microsc 1985, 138 (Pt 2):203-12
- Hufnagl P, Bayer G, Oberbamscheidt P, et al.: Comparison of different telepathology solutions for primary frozen section diagnostic. Anal Cell Pathol 2000, 21:161-7
- Husain OA, Watts KC: Preparatory methods for DNA hydrolysis, cytochemistry, immunocytochemistry and ploidy analysis. Their application to automated and routine diagnostic cytopathology. Anal Quant Cytol Histol 1987, 9:218-24
- Husain OA, Watts KC, Lorriman F, et al.: Semi-automated cervical smear pre-screening systems: an evaluation of the Cytoscan-110. Anal Cell Pathol 1993, 5:49-68
- Inoue H, Igari T, Nishikage T, et al.: A novel method of virtual histopathology using laser-scanning confocal microscopy in-vitro with untreated fresh specimens from the gastrointestinal mucosa. Endoscopy 2000, 32:439-43
- Jakubovic HR: A critique of the American and Canadian procedures of examination in dermatopathology. Am J Dermatopathol 1982, 4:283-4
- Jancarkova N, Zikan M, Pohlreich P, et al.: [Detection and occurrence of BRCA 1 gene mutation in patients with carcinoma of the breast and ovary]. Ceska Gynekol 2003, 68:11-6
- Johnson CA, Cioffi GA, Van Buskirk EM: Frequency doubling technology perimetry using a 24--2 stimulus presentation pattern. Optom Vis Sci 1999, 76:571-81
- Johnston W, Gannon D, Nitzberg B: Grids as production computing environments: The engineering aspects of NASA's information power Grid. Eighth IEEE International Symposium on High Performance Distributed Computing, Redondo Beach, CA, August 1999 1999, IEEE Computer Society Press: Los Alamitos, CA,
- Jukic DM, Bifulco CB: Telepathology and pathology at distance: an overview. Croat Med J 1999, 40:421-4
- Kaczmarek E, Gorna A, Majewski P: Techniques of image analysis for quantitative immunohistochemistry. Rocz Akad Med Bialymst 2004, 49 Suppl 1:155-8
- Kanter-Smoler G, Bjork J, Fritzell K, et al.: Novel Findings in Swedish Patients With MYH-Associated Polyposis: Mutation Detection and Clinical Characterization. Clin Gastroenterol Hepatol 2006, 4:499-506
- Karslioglu Y, Celasun B, Gunhan O: Contribution of morphometry in the differential diagnosis of fine-needle thyroid aspirates. Cytometry B Clin Cytom 2005, 65:22-8
- Kato N, Shibuya H, Fukase M, et al.: Involvement of adenomatous polyposis coli (APC) gene in testicular yolk sac tumor of infants. Hum Pathol 2006, 37:48-53
- Kayser G, Baumhakel JD, Szoke T, et al.: Vascular diffusion density and survival of patients with primary lung carcinomas. Virchows Arch 2003, 442:462-7
- Kayser G, Gerlach U, Walch A, et al.: Numerical and structural centrosome aberrations are an early and stable event in the adenoma-carcinoma sequence of colorectal carcinomas. Virchows Arch 2005, 447:61-5
- Kayser G, Kayser K: Telepathology in Europe. Virtual Microscopy and Virtual Slides in Teaching, Diagnosis and Research. Edited by J. Gu RO. Boca Raton, Taylor & Francis, 2005
- Kayser G, Radziszowski D, Bzdyl P, et al.: Theory and implementation of an electronic, automated measurement system for images obtained from immunohistochemically stained slides. Anal Quant Cytol Histol 2006, 28:27-38
- Kayser G, Riede U, Werner M, et al.: Towards an automated morphological classification of histological images of common lung carcinomas. Elec J Pathol Histol 2002, 8:022-03
- Kayser K: Logic and diagnosis. Methods Inf Med 1975, 14:76-80
- Kayser K: Application of structural pattern recognition in histopathology. Syntactic and structural pattern recognition. Edited by G. Ferraté TP, A. Sanfeliu, H. Bunke. Berlin Heidelberg New York, Springer, 1988, pp 115-35
- Kayser K: Analytical Lung Pathology. Heidelberg, new York, Springer, 1992
- Kayser K: Telepathology--visual telecommunication in pathology. An introduction. Zentralbl Pathol 1992, 138:381-2
- Kayser K: Progress in telepathology. In Vivo 1993, 7:331-3
- Kayser K: Telepathology in Europe. Its practical use. Arch Anat Cytol Pathol 1995, 43:196-9
- Kayser K: [Telemedicine]. Wien Klin Wochenschr 1996, 108:532-40
- Kayser K: Telepathology, images, and multimedia archives. Adv Clin Path 1998, 2:157
- Kayser K: Telepathology in Europe. Anal Cell Pathol 2000, 21:95-6
- Kayser K: Basic considerations on DNA cytometry. Elec J Pathol Histol 2002, 8:021-08
- Kayser K: Interdisciplinary telecommunication and expert teleconsultation in diagnostic pathology: present status and future prospects. J Telemed Telecare 2002, 8:325-30
- Kayser K, Baumgartner K, Gabius HJ: Cytometry with DAPI-stained tumor imprints. A reliable tool for improved intraoperative analysis of lung neoplasms. Anal Quant Cytol Histol 1996, 18:115-20
- Kayser K, Berthold S, Eichhorn S, et al.: Application of attributed graphs in diagnostic pathology. Anal Quant Cytol Histol 1996, 18:286-92
- Kayser K, Beyer M, Blum S, et al.: Recent developments and present status of telepathology. Anal Cell Pathol 2000, 21:101-6
- Kayser K, Beyer M, Blum S, et al.: Telecommunication--a new tool for quality assurance and control in diagnostic pathology. Folia Neuropathol 2000, 38:79-83
- Kayser K, Blum S, Beyer M, et al.: Routine DNA cytometry of benign and malignant pleural effusions by means of the remote quantitation server Euroquant: a prospective study. J Clin Pathol 2000, 53:760-4
- Kayser K, Bubenzer J: Microwave-assisted staining procedures in routine histopathology. Histochem J 1990, 22:365-70
- Kayser K, Burkhardt HU: The incidence of gastro-intestinal cancer in North Baden (West Germany) 1971--1977. J Cancer Res Clin Oncol 1979, 93:301-21
- Kayser K, Burkhardt HU, Jacob W: The regional registry of gastro-intestinal cancer North Baden (2.2 million inhabitants). Virchows Arch A Pathol Anat Histol 1978, 380:155-62
- Kayser K, Drlicek M: Visual telecommunication for expert consultation of intraoperative sections. Zentralbl Pathol 1992, 138:395-8
- Kayser K, Drlicek M, Rahn W: Aids of telepathology in intra-operative histomorphological tumor diagnosis and classification. In Vivo 1993, 7:395-8
- Kayser K, Fritz P, Drlicek M: Aspects of telepathology in routinary diagnostic work with specific emphasis on ISDN. Arch Anat Cytol Pathol 1995, 43:216-8
- Kayser K, Fritz P, Drlicek M, et al.: Expert consultation by use of telepathology--the Heidelberg experiences. Anal Cell Pathol 1995, 9:53-60
- Kayser K, Gabius HJ: Graph theory and the entropy concept in histochemistry. Theoretical considerations, application in histopathology and the combination with receptor-specific approaches. Prog Histochem Cytochem 1997, 32:1-106
- Kayser K, Gabius HJ: The application of thermodynamic principles to histochemical and morphometric tissue research: principles and practical outline with focus on the glycosciences. Cell Tissue Res 1999, 296:443-55
- Kayser K, Hoffgen H: Pattern recognition in histopathology by orders of textures. Med Inform (Lond) 1984, 9:55-9
- Kayser K, Hufnagl P, Kayser G, et al.: Stratified sampling: Basic ideas and application in pathology. Elec J Pathol Histol 1999, 5:994-08
- Kayser K, Jacinto SD, Bohm G, et al.: Application of computer-assisted morphometry to the analysis of prenatal development of human lung. Anat Histol Embryol 1997, 26:135-9
- Kayser K, Kayser C: Telepathology - aspects of social influence and quality assurance. Elec J Pathol 1996, 6:963-04
- Kayser K, Kayser G: Basic aspects of and recent developments in telepathology in Europe, with specific emphasis on quality assurance. Anal Quant Cytol Histol 1999, 21:319-28
- Kayser K, Kayser G: Virtual Microscopy and Automated Diagnosis. Virtual Microscopy and Virtual Slides in Teaching, Diagnosis and Research. Edited by Gu J, Ogilvie R. Boca Raton, Taylor & Francis, 2005
- Kayser K, Kayser G, Becker HD, et al.: Telediagnosis of transbronchial fine needle aspirations--a feasibility study. Anal Cell Pathol 2000, 21:207-12
- Kayser K, Kayser G, Hufnagl P: The concept of structural entropy: basic idea and applications. Elec J Pathol Histol 2002, 8:024-06
- Kayser K, Kayser G, Radziszowski D, et al.: From telepathology to virtual pathology institution: the new world of digital pathology. Rom J Morphol Embryol 1999, 45:3-9
- Kayser K, Kayser G, Radziszowski D, et al.: New Developments in Digital Pathology: From Telepathology to Virtual Pathology. Transformation of Helathcare with Information Technologies. Edited by Duplaga M, Zielinski K, Ingram D. Amsterdam, IOS Press, 2004, pp 61-9
- Kayser K, Kayser G, Radziszowski D, et al.: New developments in digital pathology: from telepathology to virtual pathology laboratory. Stud Health Technol Inform 2004, 105:61-9
- Kayser K, Kayser G, Schrader T, et al.: Recent developments and future aspects of telepathology in Europe. Krakow, PR Access, 2003, pp 163-74.
- Kayser K, Kiefer B, Burkhardt HU: Syntactic structure analysis of bronchus carcinomas - first results. Acta Stereol 1985, 4/2:249-53
- Kayser K, Kosjerina Z, Goldmann T, et al.: Phenotype and genotype associations of lung carcinoma with atypical adenomatoid hyperplasia, squamous cell dysplasia, and chromosome alterations in non-neoplastic bronchial mucosa. Rom J Morphol Embryol 2005, 46:5-10
- Kayser K, Kremer K, Tacke M: [DNA and MST entropy and current of entropy. New parameters of tumor biological characterization]. Zentralbl Pathol 1994, 139:427-31
- Kayser K, Markle C, Kugler C, et al.: Integrated nuclear fluorescence and expression of hormone-binding sites in malignant pleural effusions. Anal Quant Cytol Histol 2000, 22:364-72
- Kayser K, Oehmann A: Digital Lung Pathology. Heidelberg, CD, 2004
- Kayser K, Ramisch W, von Kenne H, et al.: Thesaurus and information. Med Inform (Lond) 1980, 5:155-66
- Kayser K, Richter B, Stryciak R, et al.: Parameters derived from integrated nuclear fluorescence, syntactic structure analysis, and vascularization in human lung carcinomas. Anal Cell Pathol 1997, 15:73-83
- Kayser K, Sandau K, Paul J, et al.: An approach based on two-dimensional graph theory for structural cluster detection and its histopathological application. J Microsc 1992, 165 (Pt 2):281-8
- Kayser K, Schlegel W: Pattern recognition in histo-pathology: basic considerations. Methods Inf Med 1982, 21:15-22
- Kayser K, Schwarzmann P: Aspects of standardization in telepathology. Zentralbl Pathol 1992, 138:389-92
- Kayser K, Shaver M, Modlinger F, et al.: Neighborhood analysis of low magnification structures (glands) in healthy, adenomatous, and carcinomatous colon mucosa. Pathol Res Pract 1986, 181:153-8
- Kayser K, Stute H, Bubenzer J, et al.: Combined morphometrical and syntactic structure analysis as tools for histomorphological insight into human lung carcinoma growth. Anal Cell Pathol 1990, 2:167-78
- Kayser K, Stute H, Ebert W: Analysis of branching points and minimum distance between neighboring cells in primarey bronchus carcinoma. Acta Stereol 1987, 6/III:207-12
- Kayser K, Szymas J, Weinstein RS: Telepathology: Telecommunication, Electronic Education and Publication in Pathology. Berlin, Heidelberg, New Yorck, Springer, 1999
- Kayser K, Szymas J, Weinstein RS: Telepathology and Telemedicine Communication, Electronic Education and Publication in e-Health. Berlin, Veterinärspiegel Verlag, 2005
- Kayser KK, Kiefer B, Toomes H, et al.: Analysis of adenomatous structures in histopathology. Anal Quant Cytol Histol 1987, 9:273-8
- Kellenberger E, Rodrigo J, Muller P, et al.: Comparative evaluation of eight docking tools for docking and virtual screening accuracy. Proteins 2004, 57:225-42
- Kerl H, Cerroni L, Burg G, et al.: International Board Certification in Dermatopathology: a worldwide effort to raise standards in dermatopathology. Am J Dermatopathol 2005, 27:357-9
- Kerl H, Cerroni L, Burg G, et al.: International board certification in dermatopathology: paving the way for the future. Am J Dermatopathol 2004, 26:439-40
- Kim DK, Yoo SK, Kim SH: Instant wireless transmission of radiological images using a personal digital assistant phone for emergency teleconsultation. J Telemed Telecare 2005, 11 Suppl 2:58-61
- King MA, Scotty N, Klein RL, et al.: Particle detection, number estimation, and feature measurement in gene transfer studies: optical fractionator stereology integrated with digital image processing and analysis. Methods 2002, 28:293-9
- Kohl H: [Certification of pathology institutions --a new direction? The view of a certification institute]. Z Arztl Fortbild Qualitatssich 2004, 98:739-43
- Kononen J, Bubendorf L, Kallioniemi A, et al.: Tissue microarrays for high-throughput molecular profiling of tumor specimens. Nat Med 1998, 4:844-7
- Kopper L, Timar J: Genomics of renal cell cancer-- does it provide breakthrough? Pathol Oncol Res 2006, 12:5-11
- Kovai L, Lonari S, Paladino J, et al.: The Croatian telemedicine. Stud Health Technol Inform 2000, 77:1146-50
- Kunze KD, Boecking A, Haroske G, et al.: Remote quantitation in the framework of telepathology. Adv Clin Path 1998, 2:141-3
- Kutsuna N, Hasezawa S: Morphometrical study of plant vacuolar dynamics in single cells using three-dimensional reconstruction from optical sections. Microsc Res Tech 2005, 68:296-306
- Lampe B, Hantschmann P, Dimpfl T: Prognostic relevance of immunohistology, tumor size and vascular space involvement in axillary node negative breast cancer. Arch Gynecol Obstet 1998, 261:139-46
- Lancaster JM, Carney ME, Futreal PA: BRCA 1 and 2--A Genetic Link to Familial Breast and Ovarian Cancer. Medscape Womens Health 1997, 2:7
- Larsen JO, Gundersen HJ, Nielsen J: Global spatial sampling with isotropic virtual planes: estimators of length density and total length in thick, arbitrarily orientated sections. J Microsc 1998, 191:238-48
- Laurini R, Thompson D: Fundamentals of spatial information systems. London, Academic Press, 1992
- Lee E, Chae Y, Kim I, et al.: Prognostic relevance of immunohistochemically detected lymph node micrometastasis in patients with gastric carcinoma. Cancer 2002, 94:2867-73
- Lee ES, Kim IS, Choi JS, et al.: Accuracy and reproducibility of telecytology diagnosis of cervical smears. A tool for quality assurance programs. Am J Clin Pathol 2003, 119:356-60
- Leong AS, Dale BM, Liew SH, et al.: Node-based T cell lymphoma. The clinical, immunological and morphological spectrum. Pathology 1981, 13:79-95
- Leong AS, Leong FJ: What we need to know about cancer genes. Diagn Cytopathol 1998, 18:33-40
- Leong AS, Sormunen RT, Vinyuvat S, et al.: Biologic markers in ductal carcinoma in situ and concurrent infiltrating carcinoma. A comparison of eight contemporary grading systems. Am J Clin Pathol 2001, 115:709-18
- Leong AS, Visinoni F, Visinoni C, et al.: An advanced digital image-capture computer system for gross specimens: a substitute for gross description. Pathology 2000, 32:131-5
- Leong F: An Automated Diagnostic system for Tubular Carcinoma of the Breast - a Model for Computer-based Diagnosis. Oxford, Oxford, 2002
- Leong FJ: Practical applications of Internet resources for cost-effective telepathology practice. Pathology 2001, 33:498-503
- Leong FJ: An automated diagnostic system for tubular carcinoma of the breast - a model for computer-based cancer diagnosis. Department of Engineering Science. Oxford, Oxford, 2002, pp 250
- Leong FJ, Graham AK, Schwarzmann P, et al.: Clinical trial of telepathology as an alternative modality in breast histopathology quality assurance. Telemed J E Health 2000, 6:373-7
- Leong FJ, Leong AS: Digital imaging applications in anatomic pathology. Adv Anat Pathol 2003, 10:88-95
- Leong FJ, Leong AS: Digital imaging in pathology: theoretical and practical considerations, and applications. Pathology 2004, 36:234-41
- Leong FJ, Leong AS: Digital photography in anatomical pathology. J Postgrad Med 2004, 50:62-9
- Leong FJ, McGee JO: Automated complete slide digitization: a medium for simultaneous viewing by multiple pathologists. J Pathol 2001, 195:508-14
- Leong FJ, Nicholson AG, McGee JO: Robotic telepathology: efficacy and usability in pulmonary pathology. J Pathol 2002, 197:211-7
- Lind AC, Bewtra C, Healy JC, et al.: Prospective peer review in surgical pathology. Am J Clin Pathol 1995, 104:560-6
- Loane M, Wootton R: A review of guidelines and standards for telemedicine. J Telemed Telecare 2002, 8:63-71
- Loane MA, Gore HE, Corbett R, et al.: Preliminary results from the Northern Ireland arms of the UK Multicentre Teledermatology Trial: effect of camera performance on diagnostic accuracy. J Telemed Telecare 1997, 3 Suppl 1:73-5
- Luo B, Wang Y, Wang XF, et al.: Correlation of Epstein-Barr virus and its encoded proteins with Helicobacter pylori and expression of c-met and c-myc in gastric carcinoma. World J Gastroenterol 2006, 12:1842-8
- Luo C, Peng Y, Santti R, et al.: COX-1 and -2 Expressions in Sex-Related Organs of Neonatally Estrogen-Treated Rats and in Activated and Nonactivated Macrophage RAW264.7 Cells with Phytoestrogen. Endocrine 2006, 29:161-8
- Mairinger T, Netzer TT, Schoner W, et al.: Pathologists' attitudes to implementing telepathology. J Telemed Telecare 1998, 4:41-6
- Mall G, Kayser K, Rossner JA: The loss of membrane images from oblique sectioning of biological membranes and the availability of morphometric principles-demonstrated by the examination of heart muscle mitochondria. Mikroskopie 1977, 33:246-54
- Mall G, Reinhard H, Kayser K, et al.: An effective morphometric method for electron microscopic studies on papillary muscles. Virchows Arch A Pathol Anat Histol 1978, 379:219-28
- Mandelbrot BB: The Fractal Geometry of Nature. New York, Freeman, 1982
- Maraki D, Becker J, Boecking A: Cytologic and DNA-cytometric very early diagnosis of oral cancer. J Oral Pathol Med 2004, 33:398-404
- Maraki D, Hengge UR, Becker J, et al.: Very early cytological and DNA-cytometric diagnosis of in situ carcinoma in an immunosuppressed liver transplant recipient. J Oral Pathol Med 2006, 35:58-60
- Maraki D, Yalcinkaya S, Pomjanski N, et al.: Cytologic and DNA-cytometric examination of oral lesions in lichen planus. J Oral Pathol Med 2006, 35:227-32
- Mariuzzi L, Mombello A, Rucco V, et al.: Quantitative study of ductal breast cancer progression: signatures of nuclei in proliferating breast lesions and in situ cancer. Adv Clin Path 2000, 4:87-97
- Marsan C, Vacher-Lavenu MC: Telepathology: a tool to aid in diagnosis and quality assurance in cervicovaginal cytology. Cytopathology 1995, 6:339-42
- Marsan C, Vacher-Lavenu MC, Cochand-Priollet B: A cytotelepathology consulting station. Pol J Pathol 1998, 49:41-2
- Martinez-Arribas F, Martin-Garabato E, Lafuente P, et al.: Proliferation measurement in breast cancer by two different methods. Anticancer Res 2006, 26:199-202
- Mathieu O, Cruz-Orive LM, Hoppeler H, et al.: Measuring error and sampling variation in stereology: comparison of the efficiency of various methods for planar image analysis. J Microsc 1981, 121:75-88
- Matousek J: Geometric range searching. ACM Comput Surveys 1994, 26:421-61
- Mattfeldt T, Frey H, Rose C: Second-order stereology of benign and malignant alterations of the human mammary gland. J Microsc 1993, 171 (Pt 2):143-51
- Mattfeldt T, Gottfried H, Schmidt V, et al.: Classification of spatial textures in benign and cancerous glandular tissues by stereology and stochastic geometry using artificial neural networks. J Microsc 2000, 198 (Pt 2):143-58
- Mattfeldt T, Mall G, Gharehbaghi H, et al.: Estimation of surface area and length with the orientator. J Microsc 1990, 159 (Pt 3):301-17
- Mattfeldt T, Vogel U, Gottfried HW: [Three-dimensional spatial texture of adenocarcinoma of the prostate by a combination of stereology and digital image analysis]. Verh Dtsch Ges Pathol 1993, 77:73-7
- Mayhew TM: Second-order stereology and ultrastructural examination of the spatial arrangements of tissue compartments within glomeruli of normal and diabetic kidneys. J Microsc 1999, 195 (Pt 2):87-95
- Mayhew TM, Burton GJ: Stereology and its impact on our understanding of human placental functional morphology. Microsc Res Tech 1997, 38:195-205
- Mescoli C, Rugge M, Pucciarelli S, et al.: High prevalence of isolated tumor cells in regional lymph nodes from PN0 colorectal cancer. J Clin Pathol 2006
- Mikel UV, Fishbein WN, Bahr GF: Some practical considerations in quantitative absorbance microspectrophotometry. Preparation techniques in DNA cytophotometry. Anal Quant Cytol Histol 1985, 7:107-18
- Mireskandari M, Kayser G, Hufnagl P, et al.: Teleconsultation in diagnostic pathology: experience from Iran and Germany with the use of two European telepathology servers. Journal of Telemedicine and Telecare 2004, 10:99-103
- Mohsin S, Taylor D, Lee TC: Three-dimensional reconstruction of Haversian systems in ovine compact bone. Eur J Morphol 2002, 40:309-15
- Molnar B, Berczi L, Diczhazy C, et al.: Digital slide and virtual microscopy based routine and telepathology evaluation of routine gastrointestinal biopsy specimens. J Clin Pathol 2003, 56:433-8
- Montironi R, Santinelli A, Pomante R, et al.: Inference network-based analyses of the histopathological effects of androgen deprivation on prostate cancer. J Pathol 1999, 187:462-8
- Mosca A, Berruti A, Russo L, et al.: The neuroendocrine phenotype in prostate cancer: basic and clinical aspects. J Endocrinol Invest 2005, 28:141-5
- Muda AO, Paradisi M, Angelo C, et al.: Three-dimensional distribution of basement membrane components in dystrophic recessive epidermolysis bullosa. J Pathol 1996, 179:427-31
- Myers DE, Anderson LD, Seifert RP, et al.: Noninvasive method for measuring local hemoglobin oxygen saturation in tissue using wide gap second derivative near-infrared spectroscopy. J Biomed Opt 2005, 10:034017
- Natiello M, Samuelson D: Three-dimensional reconstruction of the angioarchitecture of the ciliary body of the West Indian manatee (Trichechus manatus). Vet Ophthalmol 2005, 8:367-73
- Nedzved A, Ablameyko S, Pitas I: Morphological Segmentation of Histology Cell Images. 15th International Conference on Pattern Recognition (ICPR'00) 2000, 1:1500
- Nehal KS, Busam KJ, Halpern AC: Use of dynamic telepathology in Mohs surgery: a feasibility study. Dermatol Surg 2002, 28:422-6
- Netzer TT, Gschwendtner A, Schoner W, et al.: Telepathology in Austria - preliminary results of a survey amaong pathologists in Austria. Elec J Pathol 1996, 6:963-02
- Nocito A, Kononen J, Kallioniemi OP, et al.: Tissue microarrays (TMAs) for high-throughput molecular pathology research. Int J Cancer 2001, 94:1-5
- Nordrum I, Eide TJ: Remote frozen section service in Norway. Arch Anat Cytol Pathol 1995, 43:253-6
- Nordrum I, Engum B, Rinde E, et al.: Remote frozen section service: a telepathology project in northern Norway. Hum Pathol 1991, 22:514-8
- Nordrum I, Isaksen V, Arvola L: Breast carcinoma diagnosed by telepathology. J Telemed Telecare 1997, 3:172-3
- Nordrum I, Isaksen V, Johansen M, et al.: [Inaccuracy about telepathology from the Radiumhospital]. Tidsskr Nor Laegeforen 2000, 120:2939
- Nordrum II: Real-time diagnoses in telepathology. Adv Clin Path 1998, 2:127-31
- NSF T-G: NSF Tera-Grid. http://wwwteraGridorg/ 2006
- Nyquist H: Certain Topics in Telegraph Transmission Theory. reprint Proc IEEE, Vol 90, No 2, (Feb 2002) 1928, 47:617-44
- O'Brien MJ, Takahashi M, Brugal G, et al.: Digital imagery/telecytology. International Academy of Cytology Task Force summary. Diagnostic Cytology Towards the 21st Century: An International Expert Conference and Tutorial. Acta Cytol 1998, 42:148-64
- O'Callaghan JF: An alternative definition for neighborhood of a point. IEEE TRans Comput 1975, 24:1121-5
- Oberholzer M, Bianchi L, Dalquen P, et al.: Stereology in the extraction of information from images. Anal Quant Cytol Histol 1985, 7:197-204
- Oberholzer M, Christen H, Haroske G, et al.: Modern telepathology: a distributed system with open standards. Curr Probl Dermatol 2003, 32:102-14
- Oberholzer M, Fischer HR, Christen H, et al.: Telepathology: frozen section diagnosis at a distance. Virchows Arch 1995, 426:3-9
- Odze RD, Goldblum J, Noffsinger A, et al.: Interobserver variability in the diagnosis of ulcerative colitis-associated dysplasia by telepathology. Mod Pathol 2002, 15:379-86
- Ogilvie R, Groscurth P, Rohr H, et al.: WebMic, a Virtual Microscope for Learning Histology: Results of Implementation in a Medical Histology Course. Virtual Microscopy and Virtual Slides in Teaching, Diagnosis, and Research. Edited by Gu J, Ogilvie RW. Boca Raton, Taylor & Francis, 2005, pp 161-76
- Okada K, Shimura T, Suehiro T, et al.: Reduced galectin-3 expression is an indicator of unfavorable prognosis in gastric cancer. Anticancer Res 2006, 26:1369-76
- Oliveira IC, Oliveira JL, Sanchez JP, et al.: Grid requirements for the integration of biomedical information resources for health applications. Methods Inf Med 2005, 44:161-7
- Onguru O, Celasun B: Intra-hospital use of a telepathology system. Pathol Oncol Res 2000, 6:197-201
- Oram A: Peer-to-Peer: Harnessing the Power of Disruptive Technologies., O'Reilly Press: U.S.A., 2001
- Ormerod MG, Tribukait B, Giaretti W: Consensus report of the task force on standardisation of DNA flow cytometry in clinical pathology. DNA Flow Cytometry Task Force of the European Society for Analytical Cellular Pathology. Anal Cell Pathol 1998, 17:103-10
- Ormiston W: Hereditary breast cancer. Eur J Cancer Care (Engl) 1996, 5:13-20
- Pak HS: Teledermatology and teledermatopathology. Semin Cutan Med Surg 2002, 21:179-89
- Pare G, Lepanto L, Aubry D, et al.: Toward a multidimensional assessment of picture archiving and communication system success. Int J Technol Assess Health Care 2005, 21
- Paschalis EP, DiCarlo E, Betts F, et al.: FTIR microspectroscopic analysis of human osteonal bone. Calcif Tissue Int 1996, 59:480-7
- Pearson K: On lines and planes of closest fit to systems of points in space. Philosophical Magazine 1901, 2:259-572
- Petrovichev NN: [Basic directions in pathologoanatomic diagnosis of human tumors]. Vestn Ross Akad Med Nauk 2001:33-8
- Phillips KL, Anderson L, Gahm T, et al.: Quantitative DNA analysis: a comparison of conventional DNA ploidy analysis and teleploidy. Arch Anat Cytol Pathol 1995, 43:288-95
- Phillips LA, Phillips KL, Gahm T, et al.: Quantitative DNA ploidy analysis of breast carcinoma: a study of the effects of joint photographer experts group (JPEG) compression on DNA ploidy images. Diagn Cytopathol 1996, 15:231-6
- Phillips PK, Voak D, Smith K, et al.: The illusion of quality in quality management systems: meaningful accreditation. Transfus Med 1994, 4:179-83
- Planz B, Synek C, Deix T, et al.: Diagnosis of bladder cancer with urinary cytology, immunocytology and DNA-image-cytometry. Anal Cell Pathol 2001, 22:103-9
- Planz B, Synek C, Robben J, et al.: Diagnostic accuracy of DNA image cytometry and urinary cytology with cells from voided urine in the detection of bladder cancer. Urology 2000, 56:782-6
- Plinkert PK, Plinkert B, Fuchs M, et al.: [Telemedicine in otorhinolaryngology exemplified by a Tubingen-Leipzig video conference]. Hno 2000, 48:728-34
- Polcharoen W, Pilch BZ, Jakobiec FA: Frozen-section diagnosis in ophthalmic surgery. Int Ophthalmol Clin 1999, 39:195-211
- Pollice L: [New biotechnology frontiers in histocytopathology]. Recenti Prog Med 1996, 87:549-54
- Prahlow JA, Lantz PE: Medical examiner/death investigator training requirements in state medical examiner systems. J Forensic Sci 1995, 40:55-8
- Prewitt J: Object enhancement and extraction. Picture processing and Psycopictories. Edited by L Lipkin AR. New York, Academic Press, 1970, pp 75 - 149
- Raab SS, Robinson RA, Snider TE, et al.: Telepathologic review: utility, diagnostic accuracy, and interobserver variability on a difficult case consultation service. Mod Pathol 1997, 10:630-5
- Raab SS, Zaleski MS, Thomas PA, et al.: Telecytology: diagnostic accuracy in cervical-vaginal smears. Am J Clin Pathol 1996, 105:599-603
- Rabbani M, Joshi R: An overview of the JPEG 2000 still image compression standard. Signal Proc Image Com 2003, 17:3-48
- Ramsay AD, Gallagher PJ: Local audit of surgical pathology. 18 month's experience of peer review-based quality assessment in an English teaching hospital. Am J Surg Pathol 1992, 16:476-82
- Reid IM: Morphometric methods in veterinary pathology: a review. Vet Pathol 1980, 17:522-43
- Renshaw AA, Cartagena N, Granter SR, et al.: Agreement and error rates using blinded review to evaluate surgical pathology of biopsy material. Am J Clin Pathol 2003, 119:797-800
- Renshaw AA, Gould EW: Comparison of disagreement and error rates for three types of interdepartmental consultations. Am J Clin Pathol 2005, 124:878-82
- Renshaw AA, Wang E, Haja J, et al.: Fine-needle aspiration of papillary thyroid carcinoma: distinguishing between cases that performed well and those that performed poorly in the College of American Pathologists Nongynecologic Cytology Program. Arch Pathol Lab Med 2006, 130:452-5
- Renshaw AA, Wang E, Wilbur D, et al.: Interobserver agreement on microfollicles in thyroid fine-needle aspirates. Arch Pathol Lab Med 2006, 130:148-52
- Renshaw AA, Young ML, Jiroutek MR: How many cases need to be reviewed to compare performance in surgical pathology? Am J Clin Pathol 2003, 119:388-91
- Reuter VE: The pathology of bladder cancer. Urology 2006, 67:11-7; discussion 7-8
- Ricci MA, Callas PW, Montgomery WL: The Vermont Telemedicine Project: initial implementation phases. Telemed J 1997, 3:197-205
- Rickert CH, Filler TJ, Gullotta F: Three-dimensional reconstruction of senile plaques in Alzheimer's disease. J Microsc 1997, 186 (Pt 3):263-9
- Riley RS, Ben-Ezra JM, Massey D, et al.: The virtual blood film. Clin Lab Med 2002, 22:317-45
- Riley RS, Ben-Ezra JM, Massey D, et al.: Digital photography: a primer for pathologists. J Clin Lab Anal 2004, 18:91-128
- Robb J, Melello C, Odom C: Comparison of Cyto-Shuttle and cytocentrifuge as processing methods for nongynecologic cytology specimens. Diagn Cytopathol 1996, 14:305-9
- Robeneck H (editor): Mikroskopie in Forschung und Praxis. Weinheim, GIT Verlag, 1995
- Rodriguez-Cruz M, del Prado M, Salcedo M: [Genomic retinoblastoma perspectives: implications of tumor supressor gene RB1]. Rev Invest Clin 2005, 57:572-81
- Romer DJ, Suster S: Use of virtual microscopy for didactic live-audience presentation in anatomic pathology. Ann Diagn Pathol 2003, 7:67-72
- Rosenzvit M, Angel S, Alvarez L, et al.: A simple and economic slide micro-immunoenzymatic (micro-SIA) test for epidemiological studies of toxoplasmosis. Mem Inst Oswaldo Cruz 1994, 89:47-51
- Rossi S, Ou W, Tang D, et al.: Gastrointestinal stromal tumours overexpress fatty acid synthase. J Pathol 2006
- Rutty GN, Duerden RM, Carter N, et al.: Are coroners' necropsies necessary? A prospective study examining whether a "view and grant" system of death certification could be introduced into England and Wales. J Clin Pathol 2001, 54:279-84
- Salem AA, Douglas-Jones AG, Sweetland HM, et al.: Intraoperative evaluation of axillary sentinel lymph nodes using touch imprint cytology and immunohistochemistry. Part II. Results. Eur J Surg Oncol 2006
- Saltz JH: Digital pathology--the big picture. Hum Pathol 2000, 31:779-80
- Sampieri K, Hadjistilianou T, Mari F, et al.: Mutational screening of the RB1 gene in Italian patients with retinoblastoma reveals 11 novel mutations. J Hum Genet 2006, 51:209-16
- Sanfeliu A, Fu KS, Prewitt J: An application of distance measure between graphs to the analysis of muscle tissue pattern recognition. Saragota Springs Meeting, Proc 1981:86-9
- Sangma C, Chuakheaw D, Jongkon N, et al.: Virtual screening for anti-HIV-1 RT and anti-HIV-1 PR inhibitors from the Thai medicinal plants database: a combined docking with neural networks approach. Comb Chem High Throughput Screen 2005, 8:417-29
- Saponara R, Menditto A, Russo G, et al.: [Review of the literature on BRCA 1 and BRCA 2]. Minerva Ginecol 2001, 53:72-4
- Saunders PT: An Introduction to Catastrophe Theory. Cambridge, Cambridge University Press, 1980
- Schiffer M: Legal aspects of telepathology. Zentralbl Pathol 1992, 138:393-4
- Schnorrenberg F, Pattichis CS, Schizas CN, et al.: Content-based retrieval of breast cancer biopsy slides. Technol Health Care 2000, 8:291-7
- Schrader T, Feig T, Hufnagl P, et al.: A userfriendly Telepathology Service at the Internet – The Telepathology Consultation Center of the UICC. Elec J Pathol Histol 2003, 9:031-07
- Schulte EK, Wittekind DH: Standardization of the Feulgen reaction: the influence of chromatin condensation on the kinetics of acid hydrolysis. Anal Cell Pathol 1990, 2:149-57
- Schurmann G, Mattfeldt T, Feichter G, et al.: Stereology, flow cytometry, and immunohistochemistry of follicular neoplasms of the thyroid gland. Hum Pathol 1991, 22:179-84
- Schwarzmann P: Telemicroscopy. Design considerations for a key tool in telepathology. Zentralbl Pathol 1992, 138:383-7
- Schwarzmann P, Binder B, Kaser M, et al.: European field tests with HISTKOM telepathology equipment. Stud Health Technol Inform 1999, 64:192-207
- Schwarzmann P, Binder B, Klose R: Technical aspects of telepathology with emphasis on future development. Anal Cell Pathol 2000, 21:107-26
- Schwarzmann P, Binder B, Klose R, et al.: Histkom - evaluation of active telepathology in fieldtests. Adv Clin Path 1998, 2:135-8
- Schwarzmann P, Schmid J, Binder B, et al.: Field test to evaluate telepathology in telemedicine. J Telemed Telecare 1996, 2 Suppl 1:17-20
- Schwarzmann P, Schmid J, Schnorr C, et al.: Telemicroscopy stations for telepathology based on broadband and ISDN connections. Arch Anat Cytol Pathol 1995, 43:209-15
- Seth RM, Burger AM, Kahn HJ: Analysis of the HER2/neu gene amplification in microdissected breast cancer tumour samples. Anticancer Res 2006, 26:927-31
- Shannon C: Am mathematical theory of communication. Bell Sys Tech J 1948, 27:379-423
- Shannon C: A mathematical theory of communication. Bell Sys Tech J 1948, 27:623-56
- Shannon C: Communication in the Presence of Noise. reprint: Proc IEEE, Vol 86, No 2, (Feb 1998) 1949, 37
- Singh N, Akbar N, Sowter C, et al.: Telepathology in a routine clinical environment: implementation and accuracy of diagnosis by robotic microscopy in a one-stop breast clinic. J Pathol 2002, 196:351-5
- Singson RP, Natarajan S, Greenson JK, et al.: Virtual microscopy and the Internet as telepathology consultation tools. A study of gastrointestinal biopsy specimens. Am J Clin Pathol 1999, 111:792-5
- Slodkowska J: [Use of telepathology in histopathologic diagnosis]. Pneumonol Alergol Pol 2000, 68:407-10
- Slodkowska J, Wiatr E, Kayser K, et al.: [Telepathology in diagnostic consultations for controversial neoplastic growths in the lung--personal experience]. Pneumonol Alergol Pol 2001, 69:247-56
- Sloot PM, Boukhanovsky AV, Keulen W, et al.: A Grid-based HIV expert system. J Clin Monit Comput 2005, 19:263-78
- Smith MT, Connolly JL, Fletcher CD: Board certification in anatomic pathology. Am J Surg Pathol 2003, 27:267-8
- Sowter C, Wells CA: Telepathology: assessment of the implications and applications of telepathology for practical diagnostic pathology. J Clin Pathol 1998, 51:714-5
- Stalder M, Deller T, Staufenbiel M, et al.: 3D-Reconstruction of microglia and amyloid in APP23 transgenic mice: no evidence of intracellular amyloid. Neurobiol Aging 2001, 22:427-34
- Stauch G, Schweppe KW, Kayser K: Diagnostic errors in interactive telepathology. Anal Cell Pathol 2000, 21:201-6
- Stauch G, Schweppe KW, Poetz M: One year experience with telepathology fro frozen sections. Elec J Pathol 1995, 4:954-08
- Steffen B, Gianom D, Winkler C, et al.: [Frozen section diagnosis using telepathology]. Swiss Surg 1997, 3:25-9
- Stewart I: The Mathematics of Chaos, Does God Play Dice? Harmondsworth, Middlesex, Penguin Books Ltd, 1989
- Sun XR, Wang J, Boecking A: [Diagnostic and prognostic use of DNA-Image-Cytometry in cervical squamous intraepithelial lesions and invasive carcinoma]. Zhonghua Bing Li Xue Za Zhi 2005, 34:435-7
- Sutherland JV, van den Heuvel WJ, Ganous T, et al.: Towards an intelligent hospital environment: or of the future. Stud Health Technol Inform 2005, 118:278-312
- Suthipintawong C, Leong AS, Chan KW, et al.: Immunostaining of estrogen receptor, progesterone receptor, MIB1 antigen, and c-erbB-2 oncoprotein in cytologic specimens: a simplified method with formalin fixation. Diagn Cytopathol 1997, 17:127-33
- Sychra JJ, Bartels PH, Taylor J, et al.: Cytoplasmic and nuclear shape analysis for computerized cell recognition. Acta Cytol 1976, 20:68-78
- Szymas J: Teleeducation and telepathology for open and distance education. Anal Cell Pathol 2000, 21:183-91
- Szymas J: Telepathology, an innovative tool for versatile education and training in diagnostic pathology. Elec J Pathol 2002, 8:021-07
- Szymas J, Papierz W, Danilewicz M: Real-time teleneuropathology for a second opinion of neurooncological cases. Folia Neuropathol 2000, 38:43-6
- Szymas J, Wolf G: Telepathology by the internet. Adv Clin Path 1998, 2:133-5
- Szymas J, Wolf G: Remote microscopy through the internet. Pol J Pathol 1999, 50:37-42
- Szymas J, Wolf G, Papierz W, et al.: Online Internet-based robotic telepathology in the diagnosis of neuro-oncology cases: a teleneuropathology feasibility study. Hum Pathol 2001, 32:1304-8
- Takasaki S, Hano H: Three-dimensional observations of the human hepatic artery (Arterial system in the liver). J Hepatol 2001, 34:455-66
- Talve L, Kainu J, Collan Y, et al.: Immunohistochemical expression of p53 protein, mitotic index and nuclear morphometry in primary malignant melanoma of the skin. Pathol Res Pract 1996, 192:825-33
- Tamura H, More S, Yamawaki T: Texture features corresponding to visual perception. IEEE Trans on Systems, Man, and Cybernetics (SMC) 1978, 8:460-73
- Tan DS, Potts HW, Leong AC, et al.: The biological and prognostic significance of cell polarity and E-cadherin in grade I infiltrating ductal carcinoma of the breast. J Pathol 1999, 189:20-7
- Tan LP, Ng BK, Balraj P, et al.: Gene symbol: APC. Disease: colorectal cancer. Hum Genet 2005, 118:545
- Tanaka N, Ueno T, Ikeda H, et al.: CYBEST model 3 automated cytologic screening system for uterine cancer utilizing image analysis processing. Anal Quant Cytol 1982, 4:279-85
- Tao L, Lefevre M, Ricci S, et al.: Detection of occult carcinomatous diffusion in lymph nodes from head and neck squamous cell carcinoma using real-time RT-PCR detection of cytokeratin 19 mRNA. Br J Cancer 2006, 94:1164-9
- Tassarow L: Wie der Zufall will? Heidelberg, Spektrum, 1998
- Thompson D, Bartels PH, Bartels HG, et al.: Knowledge-guided histometry of the basal cell layer in prostatic intraepithelial neoplasia. Anal Quant Cytol Histol 1996, 18:177-84
- Tiehan L, Burak O, Wayne W: A real-time background subtraction method with camera motion compensation. Proceedings, International Conference on Multimedia and Exhibition, IEEE 2004
- Tomakidi P, Koke U, Kern R, et al.: Assessment of acute cyto- and genotoxicity of corrosion eluates obtained from orthodontic materials using monolayer cultures of immortalized human gingival keratinocytes. J Orofac Orthop 2000, 61:2-19
- Trogadis JE, Ng GY, O'Dowd BF, et al.: Dopamine D1 receptor distribution in Sf9 cells imaged by confocal microscopy: a quantitative evaluation. J Histochem Cytochem 1995, 43:497-506
- Troxel D: Error in Surgical Pathology. Am J Pathol 2004, 28:1092-5
- Tsuchihashi Y, Mazaki T, Murata S, et al.: Telepathology and Cytology in Kyoto, JAPAN, to Support Regional Medicine, with Special References to Their Need, Accuracy and Cost. Adv Clin Path 1998, 2:131-2
- Tsuchihashi Y, Mazaki T, Nakasato K, et al.: The basic diagnostic approaches used in robotic still-image telepathology. J Telemed Telecare 1999, 5 Suppl 1:S115-7
- Tsuchihashi Y, Okada Y, Ogushi Y, et al.: The current status of medicolegal issues surrounding telepathology and telecytology in Japan. J Telemed Telecare 2000, 6 Suppl 1:S143-5
- Tucker JH, Busch C, Spatz A, et al.: An experimental inter-expert telepathology network using static imaging. J Clin Pathol 2001, 54:752-7
- Turnbull C, Hodgson S: Genetic predisposition to cancer. Clin Med 2005, 5:491-8
- Ueda K: [Certified clinical pathologists and the program of postgraduate training in clinical pathology]. Rinsho Byori 1994, 42:504-7
- van den Tweel JG: [Autopsies as an important indicator for quality control]. Ned Tijdschr Geneeskd 1999, 143:2351-4
- Van der Laak J, Pahlplatz M, Hanselaar A, et al.: Hue-saturation-density (HSD) model for stain recognition in digitized images from transmitted light microscopy. Cytometry 2000, 52:275-84
- van Diest PJ, Kayser K, Meijer GA, et al.: Syntactic structure analysis. Pathologica 1995, 87:255-62
- Veronesi A, de Giacomi C, Magri MD, et al.: Familial breast cancer: characteristics and outcome of BRCA 1-2 positive and negative cases. BMC Cancer 2005, 5:70
- Virchow R: Cellular Pathologie. Virchows Arch 1855, 8:3-39
- Voronoi G: Nouvelles applications des paramêtres continus à la théorie des formes quadratiques, dexièmes memoire: recherches sur les parallèloedres primitifs. J Reine Angew Math 1902, 134:188-287
- Voss K, Süße H: Praktische Bildverarbeitung. München, Wien, Carl Hanser Verlag, 1991
- Wakely SL, Baxendine-Jones JA, Gallagher PJ, et al.: Aberrant diagnoses by individual surgical pathologists. Am J Surg Pathol 1998, 22:77-82
- Walter GF: Teleneuropathology: a means to improve the correctness of neuropathological diagnoses in clinical practice. Critical Reviews in Neurosurgery 1999, 9:1-11
- Walter GF, Matthies HK, Brandis A, et al.: Telemedicine of the future: teleneuropathology. Technol Health Care 2000, 8:25-34
- Wan WH, Fortuna MB, Furmanski P: A rapid and efficient method for testing immunohistochemical reactivity of monoclonal antibodies against multiple tissue samples simultaneously. J Immunol Methods 1987, 103:121-9
- Watanabe T, Komuro Y, Kiyomatsu T, et al.: Prediction of sensitivity of rectal cancer cells in response to preoperative radiotherapy by DNA microarray analysis of gene expression profiles. Cancer Res 2006, 66:3370-4
- Wayne W, Burak O, Tiehan L: Smart cameras as embedded systems. IEEE Computer 2002, 35:48-53
- Wearne SL, Rodriguez A, Ehlenberger DB, et al.: New techniques for imaging, digitization and analysis of three-dimensional neural morphology on multiple scales. Neuroscience 2005, 136:661-80
- Weibel ER: Stereological methods in cell biology: where are we--where are we going? J Histochem Cytochem 1981, 29:1043-52
- Weibel ER: Design of biological organisms and fractal goemetry. Fractals in Biology and Medicine. Edited by Nonnenmacher TF, Losa GA, Weibel ER. Basel, Borkhäuser, 1993, pp 68-85
- Weichert W, Ullrich A, Schmidt M, et al.: Expression patterns of polo-like kinase 1 in human gastric cancer. Cancer Sci 2006, 97:271-6
- Weinberg DS, Allaert FA, Dusserre P, et al.: Telepathology diagnosis by means of digital still images: an international validation study. Hum Pathol 1996, 27:111-8
- Weinstein LJ, Epstein JI, Edlow D, et al.: Static image analysis of skin specimens: the application of telepathology to frozen section evaluation. Hum Pathol 1997, 28:30-5
- Weinstein MH, Epstein JI: Telepathology diagnosis of prostrate needle biopsies. Hum Pathol 1997, 28:22-9
- Weinstein RS: Prospects for telepathology. Hum Pathol 1986, 17:433-4
- Weinstein RS: Telepathology comes of age in Norway. Hum Pathol 1991, 22:511-3
- Weinstein RS: Static image telepathology in perspective. Hum Pathol 1996, 27:99-101
- Weinstein RS: Innovations in medical imaging and virtual microscopy. Hum Pathol 2005, 36:317-9
- Weinstein RS, Bhattacharyya A, Yu YP, et al.: Pathology consultation services via the Arizona-International Telemedicine Network. Arch Anat Cytol Pathol 1995, 43:219-26
- Weinstein RS, Bhattacharyya AK, Graham AR, et al.: Telepathology: a ten-year progress report. Hum Pathol 1997, 28:1-7
- Weinstein RS, Bloom KJ, Rozek LS: Telepathology and the networking of pathology diagnostic services. Arch Pathol Lab Med 1987, 111:646-52
- Weinstein RS, Bloom KJ, Rozek LS: Telepathology. Long-distance diagnosis. Am J Clin Pathol 1989, 91:S39-42
- Weinstein RS, Decour MR, Liang C, et al.: Reinventation of light microscopy: array microscopy and ultrarapidly scanned virtual slides for diagnostic pathology and education. Virtual Microscopy and Virtual slides in Teaching, Diagnosis, and Research. Edited by Gu J, Ogilvie R, edts. Boca Raton, Taylor & Francis, 2005
- Weinstein RS, Descour MR, Liang C, et al.: An array microscope for ultrarapid virtual slide processing and telepathology. Design, fabrication, and validation study. Hum Pathol 2004, 35:1303-14
- Weinstein RS, Descour MR, Liang C, et al.: Telepathology overview: from concept to implementation. Hum Pathol 2001, 32:1283-99
- Weisz-Carrington P, Blount M, Kipreos B, et al.: Telepathology between Richmond and Beckley Veterans Affairs Hospitals: report on the first 1000 cases. Telemed J 1999, 5:367-73
- Wellnitz U, Binder B, Fritz P, et al.: Reliability of telepathology for frozen section service. Anal Cell Pathol 2000, 21:213-22
- Wellnitz U, Fritz P, Voudouri V, et al.: The validity of telepathological frozen section diagnosis with ISDN-mediated remote microscopy. Virchows Arch 2000, 437:52-7
- Werle B, Kraft C, Lah TT, et al.: Cathepsin B in infiltrated lymph nodes is of prognostic significance for patients with nonsmall cell lung carcinoma. Cancer 2000, 89:2282-91
- Werle B, Lotterle H, Schanzenbacher U, et al.: Immunochemical analysis of cathepsin B in lung tumours: an independent prognostic factor for squamous cell carcinoma patients. Br J Cancer 1999, 81:510-9
- Werner M, Georgii A, Bernhards J, et al.: Characterization of giant cells in Hodgkin's lymphomas by immunohistochemistry applied to randomly collected diagnostic biopsies from the German Hodgkin Trial. Hematol Oncol 1990, 8:241-50
- Wheeless LL, Badalament RA, de Vere White RW, et al.: Consensus review of the clinical utility of DNA cytometry in bladder cancer.Report of the DNA Cytometry Consensus Conference. Cytometry 1993, 14:478-81
- Whittaker M: The Fourier theory of the cardinal functions. Edingburgh, 1929
- Wied GL: Quality assurance measures in cytopathology. J Reprod Med 1988, 33:495
- Wied GL, Bartels PH, Bibbo M, et al.: Computer-assisted quality assurance. Acta Cytol 1996, 40:1-3
- Wikenheiser-Brokamp KA: Retinoblastoma family proteins: insights gained through genetic manipulation of mice. Cell Mol Life Sci 2006
- Wilcox-Honnold PM: Breast cancer and gene testing: risk, rationale, and responsibilities of primary care providers. Lippincotts Prim Care Pract 1998, 2:271-83
- Wilkinson DS: The role of technology in the clinical laboratory of the future. Clin Lab Manage Rev 1997, 11:322-30
- Williams BH: The AFIP center for telemedicine application--pathology for the twenty-first century. Telemed Virtual Real 1998, 3:64-5
- Williams BH, Mullick FG, Becker RL, et al.: A national treasure goes online: the Armed Forces Institute of Pathology. MD Comput 1998, 15:260-5
- Williams BH, Mullick FG, Butler DR, et al.: Clinical evaluation of an international static image-based telepathology service. Hum Pathol 2001, 32:1309-17
- Woenckhaus J, Franke FE, Hackethal A, et al.: Glucocorticosteroid receptors in ovarian carcinomas. Oncol Rep 2006, 15:1137-40
- Wright LD, Jr., Pillinger CL: Networking pathology services: adjusting to managed care. Clin Lab Med 1996, 16:227-41
- Xiao X, Ni LM: Internet QoS - A big picture. IEEE Network 1999, 13:8-18
- Xu Y, Sugiyama H: Formation of the G-quadruplex and i-motif structures in retinoblastoma susceptibility genes (Rb). Nucleic Acids Res 2006, 34:949-54
- Yagi Y: Telepathology support for the Mediterranean Institute for Transplantation and Advanced Specialized Therapies. Telemed Today 2000, 8:6, 16-7
- Yu P, Hilton P: Work practice changes caused by the introduction of a picture archiving and communication system. J Telemed Telecare 2005, 11 Suppl 2:104-7
- Zahed L, Murer-Orlando M, Bobrow M: The application of automated metaphase scanning to direct preparations of chorionic villi. Prenat Diagn 1989, 9:7-17
- Zahn C: Graph-theoretical methods for detecting and describing graph clusters. IEEE Trans Comput 1971, C-20:68-86
- Zaitoun AM, Al Mardini H, Awad S, et al.: Quantitative assessment of fibrosis and steatosis in liver biopsies from patients with chronic hepatitis C. J Clin Pathol 2001, 54:461-5
- Zarbo RJ, Meier FA, Raab SS: Error detection in anatomic pathology. Arch Pathol Lab Med 2005, 129:1237-45
- Zhao W, Olshefski D, Schulzrinne H: Internet quality of service: An overview. Technical Report, Columbia Univ, New York 2000, CUCS-003-00
- Zhou J, Hogarth MA, Walters RF, et al.: Hybrid system for telepathology. Hum Pathol 2000, 31:829-33
- Ziol M, Vacher-Lavenu MC, Heudes D, et al.: Expert consultation for cervical carcinoma smears. Reliability of selected-field videomicroscopy. Anal Quant Cytol Histol 1999, 21:35-41
- Zito FA, Marzullo F, D'Errico D, et al.: Quicktime virtual reality technology in light microscopy to support medical education in pathology. Mod Pathol 2004, 17:728-31